Open Access
ARTICLE
DeBERTa-GRU: Sentiment Analysis for Large Language Model
1 Department of Informatics for Business, College of Business, King Khalid University, Abha, 61421, Saudi Arabia
2 Department of Computer Science, College of Computer Engineering and Sciences, Prince Sattam bin Abdulaziz University, Al-Kharj, 11942, Saudi Arabia
3 School of Electrical and Information Engineering, Zhengzhou University, Zhengzhou, 450001, China
4 Department of Computer Science and Technology, Beijing University of Chemical Technology, Beijing, 100029, China
5 Department of Computer Science, Government College University Faisalabad, Faisalabad, Punjab, 38000, Pakistan
* Corresponding Authors: Abdu Gumaei. Email: ; Faisal Mehmood. Email:
(This article belongs to the Special Issue: Advance Machine Learning for Sentiment Analysis over Various Domains and Applications)
Computers, Materials & Continua 2024, 79(3), 4219-4236. https://doi.org/10.32604/cmc.2024.050781
Received 17 February 2024; Accepted 03 April 2024; Issue published 20 June 2024
Abstract
Modern technological advancements have made social media an essential component of daily life. Social media allow individuals to share thoughts, emotions, and ideas. Sentiment analysis plays the function of evaluating whether the sentiment of the text is positive, negative, neutral, or any other personal emotion to understand the sentiment context of the text. Sentiment analysis is essential in business and society because it impacts strategic decision-making. Sentiment analysis involves challenges due to lexical variation, an unlabeled dataset, and text distance correlations. The execution time increases due to the sequential processing of the sequence models. However, the calculation times for the Transformer models are reduced because of the parallel processing. This study uses a hybrid deep learning strategy to combine the strengths of the Transformer and Sequence models while ignoring their limitations. In particular, the proposed model integrates the Decoding-enhanced with Bidirectional Encoder Representations from Transformers (BERT) attention (DeBERTa) and the Gated Recurrent Unit (GRU) for sentiment analysis. Using the Decoding-enhanced BERT technique, the words are mapped into a compact, semantic word embedding space, and the Gated Recurrent Unit model can capture the distance contextual semantics correctly. The proposed hybrid model achieves F1-scores of 97% on the Twitter Large Language Model (LLM) dataset, which is much higher than the performance of new techniques.Keywords
Cite This Article
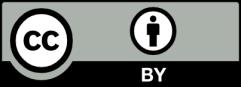
This work is licensed under a Creative Commons Attribution 4.0 International License , which permits unrestricted use, distribution, and reproduction in any medium, provided the original work is properly cited.