Open Access
ARTICLE
MSD-Net: Pneumonia Classification Model Based on Multi-Scale Directional Feature Enhancement
1 School of Computer Science and Engineering, North Minzu University, Yinchuan, 750021, China
2 School of Medical Information & Engineering, Ningxia Medical University, Yinchuan, 750004, China
3 Key Laboratory of Image and Graphics Intelligent Processing of State Ethnic Affairs Commission, North Minzu University, Yinchuan, 750021, China
* Corresponding Author: Yujie Guo. Email:
(This article belongs to the Special Issue: Deep Learning in Medical Imaging-Disease Segmentation and Classification)
Computers, Materials & Continua 2024, 79(3), 4863-4882. https://doi.org/10.32604/cmc.2024.050767
Received 17 February 2024; Accepted 30 April 2024; Issue published 20 June 2024
Abstract
Computer-aided diagnosis of pneumonia based on deep learning is a research hotspot. However, there are some problems that the features of different sizes and different directions are not sufficient when extracting the features in lung X-ray images. A pneumonia classification model based on multi-scale directional feature enhancement MSD-Net is proposed in this paper. The main innovations are as follows: Firstly, the Multi-scale Residual Feature Extraction Module (MRFEM) is designed to effectively extract multi-scale features. The MRFEM uses dilated convolutions with different expansion rates to increase the receptive field and extract multi-scale features effectively. Secondly, the Multi-scale Directional Feature Perception Module (MDFPM) is designed, which uses a three-branch structure of different sizes convolution to transmit direction feature layer by layer, and focuses on the target region to enhance the feature information. Thirdly, the Axial Compression Former Module (ACFM) is designed to perform global calculations to enhance the perception ability of global features in different directions. To verify the effectiveness of the MSD-Net, comparative experiments and ablation experiments are carried out. In the COVID-19 RADIOGRAPHY DATABASE, the Accuracy, Recall, Precision, F1 Score, and Specificity of MSD-Net are 97.76%, 95.57%, 95.52%, 95.52%, and 98.51%, respectively. In the chest X-ray dataset, the Accuracy, Recall, Precision, F1 Score and Specificity of MSD-Net are 97.78%, 95.22%, 96.49%, 95.58%, and 98.11%, respectively. This model improves the accuracy of lung image recognition effectively and provides an important clinical reference to pneumonia Computer-Aided Diagnosis.Keywords
Cite This Article
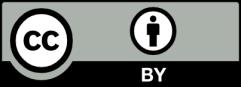
This work is licensed under a Creative Commons Attribution 4.0 International License , which permits unrestricted use, distribution, and reproduction in any medium, provided the original work is properly cited.