Open Access
ARTICLE
CNN Channel Attention Intrusion Detection System Using NSL-KDD Dataset
1 Information Systems Department, College of Computer and Information Sciences, Princess Nourah bint Abdulrahman University, Riyadh, 11671, Saudi Arabia
2 College of Computer and Information Sciences, King Saud University, Riyadh, 11362, Saudi Arabia
3 College of Computer and Information Sciences, Prince Sultan University, Riyadh, 11586, Saudi Arabia
4 Department of Educational Technologies, College of Education, Imam Abdulrahman Bin Faisal University, P.O. Box 1982, Dammam, 31441, Saudi Arabia
* Corresponding Author: Syed Umar Amin. Email:
Computers, Materials & Continua 2024, 79(3), 4319-4347. https://doi.org/10.32604/cmc.2024.050586
Received 11 February 2024; Accepted 15 April 2024; Issue published 20 June 2024
Abstract
Intrusion detection systems (IDS) are essential in the field of cybersecurity because they protect networks from a wide range of online threats. The goal of this research is to meet the urgent need for small-footprint, highly-adaptable Network Intrusion Detection Systems (NIDS) that can identify anomalies. The NSL-KDD dataset is used in the study; it is a sizable collection comprising 43 variables with the label’s “attack” and “level.” It proposes a novel approach to intrusion detection based on the combination of channel attention and convolutional neural networks (CNN). Furthermore, this dataset makes it easier to conduct a thorough assessment of the suggested intrusion detection strategy. Furthermore, maintaining operating efficiency while improving detection accuracy is the primary goal of this work. Moreover, typical NIDS examines both risky and typical behavior using a variety of techniques. On the NSL-KDD dataset, our CNN-based approach achieves an astounding 99.728% accuracy rate when paired with channel attention. Compared to previous approaches such as ensemble learning, CNN, RBM (Boltzmann machine), ANN, hybrid auto-encoders with CNN, MCNN, and ANN, and adaptive algorithms, our solution significantly improves intrusion detection performance. Moreover, the results highlight the effectiveness of our suggested method in improving intrusion detection precision, signifying a noteworthy advancement in this field. Subsequent efforts will focus on strengthening and expanding our approach in order to counteract growing cyberthreats and adjust to changing network circumstances.Keywords
Cite This Article
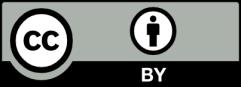
This work is licensed under a Creative Commons Attribution 4.0 International License , which permits unrestricted use, distribution, and reproduction in any medium, provided the original work is properly cited.