Open Access
ARTICLE
Exploring Motor Imagery EEG: Enhanced EEG Microstate Analysis with GMD-Driven Density Canopy Method
1 Faculty of Information Engineering and Automation, Kunming University of Science and Technology, Kunming, 650500, China
2 College of Physics and Electronic Engineering, Hanshan Normal University, Chaozhou, 521041, China
3 Department of Clinical Psychology, Second People’s Hospital of Yunnan, Kunming, 650021, China
* Corresponding Author: Jianfeng He. Email:
(This article belongs to the Special Issue: Advanced Artificial Intelligence and Machine Learning Frameworks for Signal and Image Processing Applications)
Computers, Materials & Continua 2024, 79(3), 4659-4681. https://doi.org/10.32604/cmc.2024.050528
Received 09 February 2024; Accepted 26 April 2024; Issue published 20 June 2024
Abstract
The analysis of microstates in EEG signals is a crucial technique for understanding the spatiotemporal dynamics of brain electrical activity. Traditional methods such as Atomic Agglomerative Hierarchical Clustering (AAHC), K-means clustering, Principal Component Analysis (PCA), and Independent Component Analysis (ICA) are limited by a fixed number of microstate maps and insufficient capability in cross-task feature extraction. Tackling these limitations, this study introduces a Global Map Dissimilarity (GMD)-driven density canopy K-means clustering algorithm. This innovative approach autonomously determines the optimal number of EEG microstate topographies and employs Gaussian kernel density estimation alongside the GMD index for dynamic modeling of EEG data. Utilizing this advanced algorithm, the study analyzes the Motor Imagery (MI) dataset from the GigaScience database, GigaDB. The findings reveal six distinct microstates during actual right-hand movement and five microstates across other task conditions, with microstate C showing superior performance in all task states. During imagined movement, microstate A was significantly enhanced. Comparison with existing algorithms indicates a significant improvement in clustering performance by the refined method, with an average Calinski-Harabasz Index (CHI) of 35517.29 and a Davis-Bouldin Index (DBI) average of 2.57. Furthermore, an information-theoretical analysis of the microstate sequences suggests that imagined movement exhibits higher complexity and disorder than actual movement. By utilizing the extracted microstate sequence parameters as features, the improved algorithm achieved a classification accuracy of 98.41% in EEG signal categorization for motor imagery. A performance of 78.183% accuracy was achieved in a four-class motor imagery task on the BCI-IV-2a dataset. These results demonstrate the potential of the advanced algorithm in microstate analysis, offering a more effective tool for a deeper understanding of the spatiotemporal features of EEG signals.Keywords
Cite This Article
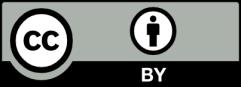