Open Access
ARTICLE
Abnormal Action Recognition with Lightweight Pose Estimation Network in Electric Power Training Scene
1 State Grid Jiangsu Electric Power Co., Ltd. Research Institute, Nanjing, 211103, China
2 School of Computer Engineering, Nanjing Institute of Technology, Nanjing, 211167, China
* Corresponding Author: Qing Yang. Email:
Computers, Materials & Continua 2024, 79(3), 4979-4994. https://doi.org/10.32604/cmc.2024.050435
Received 06 February 2024; Accepted 07 May 2024; Issue published 20 June 2024
Abstract
Electric power training is essential for ensuring the safety and reliability of the system. In this study, we introduce a novel Abnormal Action Recognition (AAR) system that utilizes a Lightweight Pose Estimation Network (LPEN) to efficiently and effectively detect abnormal fall-down and trespass incidents in electric power training scenarios. The LPEN network, comprising three stages—MobileNet, Initial Stage, and Refinement Stage—is employed to swiftly extract image features, detect human key points, and refine them for accurate analysis. Subsequently, a Pose-aware Action Analysis Module (PAAM) captures the positional coordinates of human skeletal points in each frame. Finally, an Abnormal Action Inference Module (AAIM) evaluates whether abnormal fall-down or unauthorized trespass behavior is occurring. For fall-down recognition, three criteria—falling speed, main angles of skeletal points, and the person’s bounding box—are considered. To identify unauthorized trespass, emphasis is placed on the position of the ankles. Extensive experiments validate the effectiveness and efficiency of the proposed system in ensuring the safety and reliability of electric power training.Keywords
Cite This Article
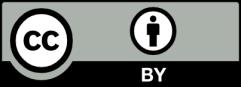
This work is licensed under a Creative Commons Attribution 4.0 International License , which permits unrestricted use, distribution, and reproduction in any medium, provided the original work is properly cited.