Open Access
ARTICLE
Joint Modeling of Citation Networks and User Preferences for Academic Tagging Recommender System
1 Faculty of Electrical Engineering and Computer Science, Ningbo University, Ningbo, 315211, China
2 Inner Mongolia Metal Material Research Institute, Baotou, 014000, China
* Corresponding Author: Baisong Liu. Email:
Computers, Materials & Continua 2024, 79(3), 4449-4469. https://doi.org/10.32604/cmc.2024.050389
Received 05 February 2024; Accepted 17 April 2024; Issue published 20 June 2024
Abstract
In the tag recommendation task on academic platforms, existing methods disregard users’ customized preferences in favor of extracting tags based just on the content of the articles. Besides, it uses co-occurrence techniques and tries to combine nodes’ textual content for modelling. They still do not, however, directly simulate many interactions in network learning. In order to address these issues, we present a novel system that more thoroughly integrates user preferences and citation networks into article labelling recommendations. Specifically, we first employ path similarity to quantify the degree of similarity between user labelling preferences and articles in the citation network. Then, the Commuting Matrix for massive node pair paths is used to improve computational performance. Finally, the two commonalities mentioned above are combined with the interaction paper labels based on the additivity of Poisson distribution. In addition, we also consider solving the model’s parameters by applying variational inference. Experimental results demonstrate that our suggested framework agrees and significantly outperforms the state-of-the-art baseline on two real datasets by efficiently merging the three relational data. Based on the Area Under Curve (AUC) and Mean Average Precision (MAP) analysis, the performance of the suggested task is evaluated, and it is demonstrated to have a greater solving efficiency than current techniques.Keywords
Cite This Article
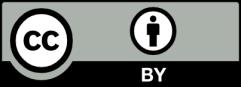