Open Access
ARTICLE
Coupling Analysis of Multiple Machine Learning Models for Human Activity Recognition
1 Department of Public Health, China Medical University, Taichung, 406040, Taiwan
2 Department of Information and Telecommunications Engineering, Ming Chuan University, Gui-Shan, Taoyuan, 333, Taiwan
3 Department of Electrical Engineering, Yuan Ze University, Chung-Li, 32003, Taiwan
4 Department and Institute of Health Service Administrations, China Medical University, Taichung, 406040, Taiwan
* Corresponding Author: Hsueh-Chun Lin. Email:
(This article belongs to the Special Issue: Advanced Artificial Intelligence and Machine Learning Frameworks for Signal and Image Processing Applications)
Computers, Materials & Continua 2024, 79(3), 3783-3803. https://doi.org/10.32604/cmc.2024.050376
Received 05 February 2024; Accepted 11 May 2024; Issue published 20 June 2024
Abstract
Artificial intelligence (AI) technology has become integral in the realm of medicine and healthcare, particularly in human activity recognition (HAR) applications such as fitness and rehabilitation tracking. This study introduces a robust coupling analysis framework that integrates four AI-enabled models, combining both machine learning (ML) and deep learning (DL) approaches to evaluate their effectiveness in HAR. The analytical dataset comprises 561 features sourced from the UCI-HAR database, forming the foundation for training the models. Additionally, the MHEALTH database is employed to replicate the modeling process for comparative purposes, while inclusion of the WISDM database, renowned for its challenging features, supports the framework’s resilience and adaptability. The ML-based models employ the methodologies including adaptive neuro-fuzzy inference system (ANFIS), support vector machine (SVM), and random forest (RF), for data training. In contrast, a DL-based model utilizes one-dimensional convolution neural network (1dCNN) to automate feature extraction. Furthermore, the recursive feature elimination (RFE) algorithm, which drives an ML-based estimator to eliminate low-participation features, helps identify the optimal features for enhancing model performance. The best accuracies of the ANFIS, SVM, RF, and 1dCNN models with meticulous featuring process achieve around 90%, 96%, 91%, and 93%, respectively. Comparative analysis using the MHEALTH dataset showcases the 1dCNN model’s remarkable perfect accuracy (100%), while the RF, SVM, and ANFIS models equipped with selected features achieve accuracies of 99.8%, 99.7%, and 96.5%, respectively. Finally, when applied to the WISDM dataset, the DL-based and ML-based models attain accuracies of 91.4% and 87.3%, respectively, aligning with prior research findings. In conclusion, the proposed framework yields HAR models with commendable performance metrics, exhibiting its suitability for integration into the healthcare services system through AI-driven applications.Graphic Abstract
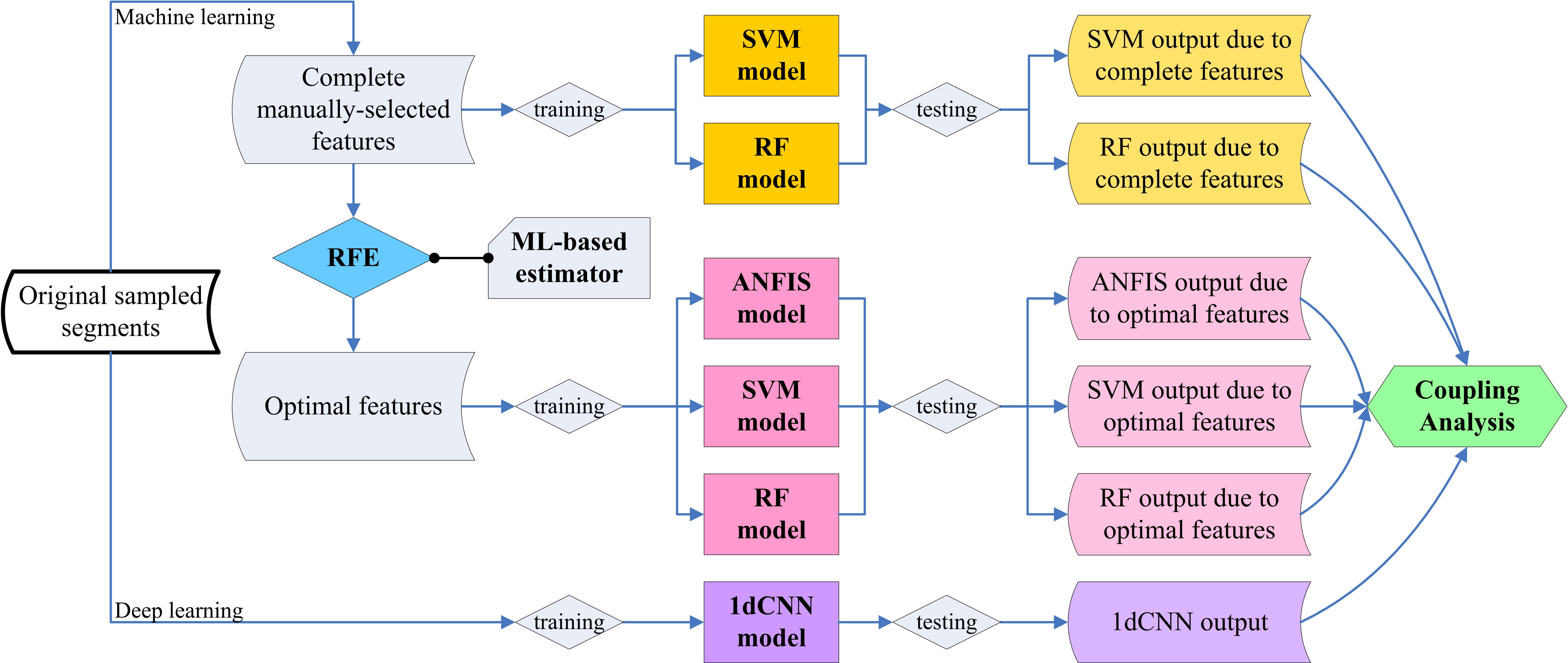
Keywords
Supplementary Material
Supplementary Material FileCite This Article
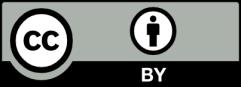
This work is licensed under a Creative Commons Attribution 4.0 International License , which permits unrestricted use, distribution, and reproduction in any medium, provided the original work is properly cited.