Open Access
ARTICLE
Predicting Users’ Latent Suicidal Risk in Social Media: An Ensemble Model Based on Social Network Relationships
1 School of Information Science and Technology, Beijing Forestry University, Beijing, 100083, China
2 Engineering Research Center for Forestry-Oriented Intelligent Information Processing of National Forestry and Grassland Administration, Beijing, 100083, China
* Corresponding Author: Chunling Wang. Email:
Computers, Materials & Continua 2024, 79(3), 4259-4281. https://doi.org/10.32604/cmc.2024.050325
Received 02 February 2024; Accepted 17 April 2024; Issue published 20 June 2024
Abstract
Suicide has become a critical concern, necessitating the development of effective preventative strategies. Social media platforms offer a valuable resource for identifying signs of suicidal ideation. Despite progress in detecting suicidal ideation on social media, accurately identifying individuals who express suicidal thoughts less openly or infrequently poses a significant challenge. To tackle this, we have developed a dataset focused on Chinese suicide narratives from Weibo’s Tree Hole feature and introduced an ensemble model named Text Convolutional Neural Network based on Social Network relationships (TCNN-SN). This model enhances predictive performance by leveraging social network relationship features and applying correction factors within a weighted linear fusion framework. It is specifically designed to identify key individuals who can help uncover hidden suicidal users and clusters. Our model, assessed using the bespoke dataset and benchmarked against alternative classification approaches, demonstrates superior accuracy, F1-score and AUC metrics, achieving 88.57%, 88.75% and 94.25%, respectively, outperforming traditional TextCNN models by 12.18%, 10.84% and 10.85%. We assert that our methodology offers a significant advancement in the predictive identification of individuals at risk, thereby contributing to the prevention and reduction of suicide incidences.Keywords
Cite This Article
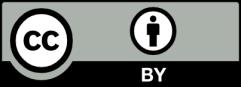