Open Access
ARTICLE
Enhancing Hyper-Spectral Image Classification with Reinforcement Learning and Advanced Multi-Objective Binary Grey Wolf Optimization
1 The WPI Business School, Worcester Polytechnic Institute, Worcester, Massachusetts, 01609-2280, USA
2 Department of Industrial Engineering, Iran University of Science and Technology, Narmak, Tehran, 16846-13114, Iran
3 ETSI de Telecomunicación, Universidad Politécnica de Madrid, Av. Complutense 30, Madrid, 28040, Spain
4 Department of Civil and Environmental Engineering, University of Maryland, College Park, Maryland, 20742, USA
5 Department of Industrial Engineering, College of Engineering, University of Houston, Houston, Texas, 77204, USA
* Corresponding Author: Diego Martín. Email:
(This article belongs to the Special Issue: Advanced Machine Learning and Optimization for Practical Solutions in Complex Real-world Systems)
Computers, Materials & Continua 2024, 79(3), 3469-3493. https://doi.org/10.32604/cmc.2024.049847
Received 19 January 2024; Accepted 09 April 2024; Issue published 20 June 2024
Abstract
Hyperspectral (HS) image classification plays a crucial role in numerous areas including remote sensing (RS), agriculture, and the monitoring of the environment. Optimal band selection in HS images is crucial for improving the efficiency and accuracy of image classification. This process involves selecting the most informative spectral bands, which leads to a reduction in data volume. Focusing on these key bands also enhances the accuracy of classification algorithms, as redundant or irrelevant bands, which can introduce noise and lower model performance, are excluded. In this paper, we propose an approach for HS image classification using deep Q learning (DQL) and a novel multi-objective binary grey wolf optimizer (MOBGWO). We investigate the MOBGWO for optimal band selection to further enhance the accuracy of HS image classification. In the suggested MOBGWO, a new sigmoid function is introduced as a transfer function to modify the wolves’ position. The primary objective of this classification is to reduce the number of bands while maximizing classification accuracy. To evaluate the effectiveness of our approach, we conducted experiments on publicly available HS image datasets, including Pavia University, Washington Mall, and Indian Pines datasets. We compared the performance of our proposed method with several state-of-the-art deep learning (DL) and machine learning (ML) algorithms, including long short-term memory (LSTM), deep neural network (DNN), recurrent neural network (RNN), support vector machine (SVM), and random forest (RF). Our experimental results demonstrate that the Hybrid MOBGWO-DQL significantly improves classification accuracy compared to traditional optimization and DL techniques. MOBGWO-DQL shows greater accuracy in classifying most categories in both datasets used. For the Indian Pine dataset, the MOBGWO-DQL architecture achieved a kappa coefficient (KC) of 97.68% and an overall accuracy (OA) of 94.32%. This was accompanied by the lowest root mean square error (RMSE) of 0.94, indicating very precise predictions with minimal error. In the case of the Pavia University dataset, the MOBGWO-DQL model demonstrated outstanding performance with the highest KC of 98.72% and an impressive OA of 96.01%. It also recorded the lowest RMSE at 0.63, reinforcing its accuracy in predictions. The results clearly demonstrate that the proposed MOBGWO-DQL architecture not only reaches a highly accurate model more quickly but also maintains superior performance throughout the training process.Keywords
Cite This Article
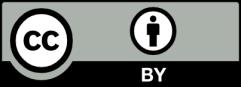
This work is licensed under a Creative Commons Attribution 4.0 International License , which permits unrestricted use, distribution, and reproduction in any medium, provided the original work is properly cited.