Open Access
ARTICLE
An Improved UNet Lightweight Network for Semantic Segmentation of Weed Images in Corn Fields
1 School of Information and Control Engineering, Jilin Institute of Chemical Technology, Jilin, 132022, China
2 School of Mechanical and Control Engineering, Baicheng Normal University, Baicheng, 137000, China
* Corresponding Author: Wenwen Li. Email:
(This article belongs to the Special Issue: Advances and Applications in Signal, Image and Video Processing)
Computers, Materials & Continua 2024, 79(3), 4413-4431. https://doi.org/10.32604/cmc.2024.049805
Received 18 January 2024; Accepted 28 March 2024; Issue published 20 June 2024
Abstract
In cornfields, factors such as the similarity between corn seedlings and weeds and the blurring of plant edge details pose challenges to corn and weed segmentation. In addition, remote areas such as farmland are usually constrained by limited computational resources and limited collected data. Therefore, it becomes necessary to lighten the model to better adapt to complex cornfield scene, and make full use of the limited data information. In this paper, we propose an improved image segmentation algorithm based on unet. Firstly, the inverted residual structure is introduced into the contraction path to reduce the number of parameters in the training process and improve the feature extraction ability; secondly, the pyramid pooling module is introduced to enhance the network’s ability of acquiring contextual information as well as the ability of dealing with the small target loss problem; and lastly, Finally, to further enhance the segmentation capability of the model, the squeeze and excitation mechanism is introduced in the expansion path. We used images of corn seedlings collected in the field and publicly available corn weed datasets to evaluate the improved model. The improved model has a total parameter of 3.79 M and miou can achieve 87.9%. The fps on a single 3050 ti video card is about 58.9. The experimental results show that the network proposed in this paper can quickly segment corn weeds in a cornfield scenario with good segmentation accuracy.Keywords
Cite This Article
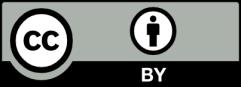