Open Access
ARTICLE
CrossLinkNet: An Explainable and Trustworthy AI Framework for Whole-Slide Images Segmentation
1 Network and Data Security Key Laboratory of Sichuan Province, University of Electronic Science and Technology of China, Chengdu, 610054, China
2 Faculty of Data Science, City University of Macau, Macau, 999078, China
* Corresponding Author: Erqiang Zhou. Email:
(This article belongs to the Special Issue: Security, Privacy, and Robustness for Trustworthy AI Systems)
Computers, Materials & Continua 2024, 79(3), 4703-4724. https://doi.org/10.32604/cmc.2024.049791
Received 17 January 2024; Accepted 23 April 2024; Issue published 20 June 2024
Abstract
In the intelligent medical diagnosis area, Artificial Intelligence (AI)’s trustworthiness, reliability, and interpretability are critical, especially in cancer diagnosis. Traditional neural networks, while excellent at processing natural images, often lack interpretability and adaptability when processing high-resolution digital pathological images. This limitation is particularly evident in pathological diagnosis, which is the gold standard of cancer diagnosis and relies on a pathologist’s careful examination and analysis of digital pathological slides to identify the features and progression of the disease. Therefore, the integration of interpretable AI into smart medical diagnosis is not only an inevitable technological trend but also a key to improving diagnostic accuracy and reliability. In this paper, we introduce an innovative Multi-Scale Multi-Branch Feature Encoder (MSBE) and present the design of the CrossLinkNet Framework. The MSBE enhances the network’s capability for feature extraction by allowing the adjustment of hyperparameters to configure the number of branches and modules. The CrossLinkNet Framework, serving as a versatile image segmentation network architecture, employs cross-layer encoder-decoder connections for multi-level feature fusion, thereby enhancing feature integration and segmentation accuracy. Comprehensive quantitative and qualitative experiments on two datasets demonstrate that CrossLinkNet, equipped with the MSBE encoder, not only achieves accurate segmentation results but is also adaptable to various tumor segmentation tasks and scenarios by replacing different feature encoders. Crucially, CrossLinkNet emphasizes the interpretability of the AI model, a crucial aspect for medical professionals, providing an in-depth understanding of the model’s decisions and thereby enhancing trust and reliability in AI-assisted diagnostics.Keywords
Cite This Article
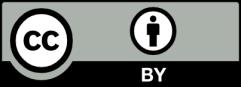