Open Access
ARTICLE
Fault Diagnosis Method of Rolling Bearing Based on MSCNN-LSTM
1 Key Laboratory of Modern Power System Simulation and Control & Renewable Energy Technology, Ministry of Education, Northeast Electric Power University, Jilin, 132012, China
2 Ministry of Education, Northeast Electric Power University, Jilin, 132012, China
* Corresponding Author: Shupeng Zheng. Email:
(This article belongs to the Special Issue: Advances and Applications in Signal, Image and Video Processing)
Computers, Materials & Continua 2024, 79(3), 4395-4411. https://doi.org/10.32604/cmc.2024.049665
Received 14 January 2024; Accepted 10 April 2024; Issue published 20 June 2024
Abstract
Deep neural networks have been widely applied to bearing fault diagnosis systems and achieved impressive success recently. To address the problem that the insufficient fault feature extraction ability of traditional fault diagnosis methods results in poor diagnosis effect under variable load and noise interference scenarios, a rolling bearing fault diagnosis model combining Multi-Scale Convolutional Neural Network (MSCNN) and Long Short-Term Memory (LSTM) fused with attention mechanism is proposed. To adaptively extract the essential spatial feature information of various sizes, the model creates a multi-scale feature extraction module using the convolutional neural network (CNN) learning process. The learning capacity of LSTM for time information sequence is then used to extract the vibration signal’s temporal feature information. Two parallel large and small convolutional kernels teach the system spatial local features. LSTM gathers temporal global features to thoroughly and painstakingly mine the vibration signal’s characteristics, thus enhancing model generalization. Lastly, bearing fault diagnosis is accomplished by using the SoftMax classifier. The experiment outcomes demonstrate that the model can derive fault properties entirely from the initial vibration signal. It can retain good diagnostic accuracy under variable load and noise interference and has strong generalization compared to other fault diagnosis models.Keywords
Cite This Article
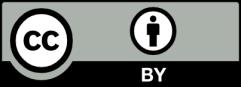