Open Access
ARTICLE
EG-STC: An Efficient Secure Two-Party Computation Scheme Based on Embedded GPU for Artificial Intelligence Systems
1 School of Computer Science, Nanjing University of Posts and Telecommunications, Nanjing, 210023, China
2 School of Computer, Nanjing University of Information Science and Technology, Nanjing, 210044, China
* Corresponding Author: Jiang Xu. Email:
(This article belongs to the Special Issue: Security, Privacy, and Robustness for Trustworthy AI Systems)
Computers, Materials & Continua 2024, 79(3), 4021-4044. https://doi.org/10.32604/cmc.2024.049233
Received 31 December 2023; Accepted 28 March 2024; Issue published 20 June 2024
Abstract
This paper presents a comprehensive exploration into the integration of Internet of Things (IoT), big data analysis, cloud computing, and Artificial Intelligence (AI), which has led to an unprecedented era of connectivity. We delve into the emerging trend of machine learning on embedded devices, enabling tasks in resource-limited environments. However, the widespread adoption of machine learning raises significant privacy concerns, necessitating the development of privacy-preserving techniques. One such technique, secure multi-party computation (MPC), allows collaborative computations without exposing private inputs. Despite its potential, complex protocols and communication interactions hinder performance, especially on resource-constrained devices. Efforts to enhance efficiency have been made, but scalability remains a challenge. Given the success of GPUs in deep learning, leveraging embedded GPUs, such as those offered by NVIDIA, emerges as a promising solution. Therefore, we propose an Embedded GPU-based Secure Two-party Computation (EG-STC) framework for Artificial Intelligence (AI) systems. To the best of our knowledge, this work represents the first endeavor to fully implement machine learning model training based on secure two-party computing on the Embedded GPU platform. Our experimental results demonstrate the effectiveness of EG-STC. On an embedded GPU with a power draw of 5 W, our implementation achieved a secure two-party matrix multiplication throughput of 5881.5 kilo-operations per millisecond (kops/ms), with an energy efficiency ratio of 1176.3 kops/ms/W. Furthermore, leveraging our EG-STC framework, we achieved an overall time acceleration ratio of 5–6 times compared to solutions running on server-grade CPUs. Our solution also exhibited a reduced runtime, requiring only 60% to 70% of the runtime of previously best-known methods on the same platform. In summary, our research contributes to the advancement of secure and efficient machine learning implementations on resource-constrained embedded devices, paving the way for broader adoption of AI technologies in various applications.Keywords
Cite This Article
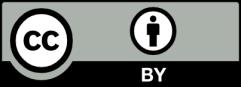