Open Access
ARTICLE
A Harmonic Approach to Handwriting Style Synthesis Using Deep Learning
1 School of Computer Science and Engineering, VIT-AP University, Amaravati, Andhra Pradesh, 522237, India
2 School of Engineering & Technology, Pimpri Chinchwad University, Pune, Maharashtra, 412106, India
3 Department of Computer Engineering, Chosun University, Gwangju, 61452, Republic of Korea
4 Independent Researcher, Amritsar, Punjab, 143001, India
* Corresponding Authors: SeongKi Kim. Email: ; Salil Bharany. Email:
Computers, Materials & Continua 2024, 79(3), 4063-4080. https://doi.org/10.32604/cmc.2024.049007
Received 25 December 2023; Accepted 20 March 2024; Issue published 20 June 2024
Abstract
The challenging task of handwriting style synthesis requires capturing the individuality and diversity of human handwriting. The majority of currently available methods use either a generative adversarial network (GAN) or a recurrent neural network (RNN) to generate new handwriting styles. This is why these techniques frequently fall short of producing diverse and realistic text pictures, particularly for terms that are not commonly used. To resolve that, this research proposes a novel deep learning model that consists of a style encoder and a text generator to synthesize different handwriting styles. This network excels in generating conditional text by extracting style vectors from a series of style images. The model performs admirably on a range of handwriting synthesis tasks, including the production of text that is out-of-vocabulary. It works more effectively than previous approaches by displaying lower values on key Generative Adversarial Network evaluation metrics, such Geometric Score (GS) (3.21 × 10) and Fréchet Inception Distance (FID) (8.75), as well as text recognition metrics, like Character Error Rate (CER) and Word Error Rate (WER). A thorough component analysis revealed the steady improvement in image production quality, highlighting the importance of specific handwriting styles. Applicable fields include digital forensics, creative writing, and document security.Keywords
Cite This Article
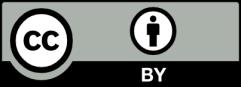
This work is licensed under a Creative Commons Attribution 4.0 International License , which permits unrestricted use, distribution, and reproduction in any medium, provided the original work is properly cited.