Open Access
ARTICLE
Fault Diagnosis Scheme for Railway Switch Machine Using Multi-Sensor Fusion Tensor Machine
1 School of Electronic and Information Engineering, Tongji University, Shanghai, 201804, China
2 Department of Architecture and Civil Engineering, City University of Hong Kong, Hong Kong, China
3 School of Computer Engineering, Jimei University, Xiamen, 361021, China
* Corresponding Author: Meng Mei. Email:
(This article belongs to the Special Issue: Industrial Big Data and Artificial Intelligence-Driven Intelligent Perception, Maintenance, and Decision Optimization in Industrial Systems)
Computers, Materials & Continua 2024, 79(3), 4533-4549. https://doi.org/10.32604/cmc.2024.048995
Received 23 December 2023; Accepted 22 April 2024; Issue published 20 June 2024
Abstract
Railway switch machine is essential for maintaining the safety and punctuality of train operations. A data-driven fault diagnosis scheme for railway switch machine using tensor machine and multi-representation monitoring data is developed herein. Unlike existing methods, this approach takes into account the spatial information of the time series monitoring data, aligning with the domain expertise of on-site manual monitoring. Besides, a multi-sensor fusion tensor machine is designed to improve single signal data’s limitations in insufficient information. First, one-dimensional signal data is preprocessed and transformed into two-dimensional images. Afterward, the fusion feature tensor is created by utilizing the images of the three-phase current and employing the CANDECOMP/PARAFAC (CP) decomposition method. Then, the tensor learning-based model is built using the extracted fusion feature tensor. The developed fault diagnosis scheme is valid with the field three-phase current dataset. The experiment indicates an enhanced performance of the developed fault diagnosis scheme over the current approach, particularly in terms of recall, precision, and F1-score.Keywords
Cite This Article
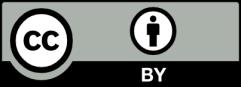
This work is licensed under a Creative Commons Attribution 4.0 International License , which permits unrestricted use, distribution, and reproduction in any medium, provided the original work is properly cited.