Open Access
ARTICLE
Developing a Model for Parkinson’s Disease Detection Using Machine Learning Algorithms
Department of Computer Science, College of Computer Science and Information System, Najran University, Najran, 55461, Saudi Arabia
* Corresponding Author: Naif Al Mudawi. Email:
(This article belongs to the Special Issue: Intelligent Detection Methods for AI-Powered Healthcare and Enhanced Medical Insights)
Computers, Materials & Continua 2024, 79(3), 4945-4962. https://doi.org/10.32604/cmc.2024.048967
Received 23 December 2023; Accepted 22 April 2024; Issue published 20 June 2024
Abstract
Parkinson’s disease (PD) is a chronic neurological condition that progresses over time. People start to have trouble speaking, writing, walking, or performing other basic skills as dopamine-generating neurons in some brain regions are injured or die. The patient’s symptoms become more severe due to the worsening of their signs over time. In this study, we applied state-of-the-art machine learning algorithms to diagnose Parkinson’s disease and identify related risk factors. The research worked on the publicly available dataset on PD, and the dataset consists of a set of significant characteristics of PD. We aim to apply soft computing techniques and provide an effective solution for medical professionals to diagnose PD accurately. This research methodology involves developing a model using a machine learning algorithm. In the model selection, eight different machine learning techniques were adopted: Namely, Random Forest (RF), Decision Tree (DT), Support Vector Machine (SVM), Naïve Bayes (NB), Light Gradient Boosting Machine (LightGBM), K-Nearest Neighbours (KNN), Extreme Gradient Boosting (XGBoost), and Logistic Regression (LR). Subsequently, the concentrated models were validated through 10-fold Cross-Validation and Receiver Operating Characteristic (ROC)—Area Under the Curve (AUC). In addition, GridSearchCV was utilised to measure each algorithm’s best parameter; eventually, the models were trained through the hyperparameter tuning approach. With 98% accuracy, LightGBM had the highest accuracy in this study. RF, KNN, and SVM came in second with 96% accuracy. Furthermore, the performance scores of NB and LR were recorded to be 76% and 83%, respectively. It is to be mentioned that after applying 10-fold cross-validation, the average performance score of LightGBM accounted for 93%. At the same time, the percentage of ROC-AUC appeared at 0.92, which indicates that this LightGBM model reached a satisfactory level. Finally, we extracted meaningful insights and figured out potential gaps on top of PD. By extracting meaningful insights and identifying potential gaps, our study contributes to the significance and impact of PD research. The application of advanced machine learning algorithms holds promise in accurately diagnosing PD and shedding light on crucial aspects of the disease. This research has the potential to enhance the understanding and management of PD, ultimately improving the lives of individuals affected by this condition.Keywords
Cite This Article
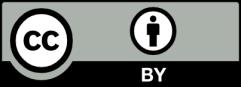
This work is licensed under a Creative Commons Attribution 4.0 International License , which permits unrestricted use, distribution, and reproduction in any medium, provided the original work is properly cited.