Open Access
ARTICLE
THAPE: A Tunable Hybrid Associative Predictive Engine Approach for Enhancing Rule Interpretability in Association Rule Learning for the Retail Sector
Information Technology Department, King Abdulaziz University, Jeddah, 21589, Saudi Arabia
* Corresponding Author: Monerah Alawadh. Email:
(This article belongs to the Special Issue: Intelligent Computing Techniques and Their Real Life Applications)
Computers, Materials & Continua 2024, 79(3), 4995-5015. https://doi.org/10.32604/cmc.2024.048762
Received 18 December 2023; Accepted 28 April 2024; Issue published 20 June 2024
Abstract
Association rule learning (ARL) is a widely used technique for discovering relationships within datasets. However, it often generates excessive irrelevant or ambiguous rules. Therefore, post-processing is crucial not only for removing irrelevant or redundant rules but also for uncovering hidden associations that impact other factors. Recently, several post-processing methods have been proposed, each with its own strengths and weaknesses. In this paper, we propose THAPE (Tunable Hybrid Associative Predictive Engine), which combines descriptive and predictive techniques. By leveraging both techniques, our aim is to enhance the quality of analyzing generated rules. This includes removing irrelevant or redundant rules, uncovering interesting and useful rules, exploring hidden association rules that may affect other factors, and providing backtracking ability for a given product. The proposed approach offers a tailored method that suits specific goals for retailers, enabling them to gain a better understanding of customer behavior based on factual transactions in the target market. We applied THAPE to a real dataset as a case study in this paper to demonstrate its effectiveness. Through this application, we successfully mined a concise set of highly interesting and useful association rules. Out of the 11,265 rules generated, we identified 125 rules that are particularly relevant to the business context. These identified rules significantly improve the interpretability and usefulness of association rules for decision-making purposes.Keywords
Cite This Article
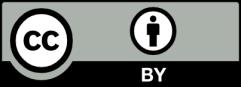
This work is licensed under a Creative Commons Attribution 4.0 International License , which permits unrestricted use, distribution, and reproduction in any medium, provided the original work is properly cited.