Open Access
ARTICLE
Scientific Elegance in NIDS: Unveiling Cardinality Reduction, Box-Cox Transformation, and ADASYN for Enhanced Intrusion Detection
Department of Information Systems College of Computer and Information Sciences, King Saud University, Riyadh, 11451, Saudi Arabia
* Corresponding Author: Amerah Alabrah. Email:
Computers, Materials & Continua 2024, 79(3), 3897-3912. https://doi.org/10.32604/cmc.2024.048528
Received 11 December 2023; Accepted 14 February 2024; Issue published 20 June 2024
Abstract
The emergence of digital networks and the wide adoption of information on internet platforms have given rise to threats against users’ private information. Many intruders actively seek such private data either for sale or other inappropriate purposes. Similarly, national and international organizations have country-level and company-level private information that could be accessed by different network attacks. Therefore, the need for a Network Intruder Detection System (NIDS) becomes essential for protecting these networks and organizations. In the evolution of NIDS, Artificial Intelligence (AI) assisted tools and methods have been widely adopted to provide effective solutions. However, the development of NIDS still faces challenges at the dataset and machine learning levels, such as large deviations in numeric features, the presence of numerous irrelevant categorical features resulting in reduced cardinality, and class imbalance in multiclass-level data. To address these challenges and offer a unified solution to NIDS development, this study proposes a novel framework that preprocesses datasets and applies a box-cox transformation to linearly transform the numeric features and bring them into closer alignment. Cardinality reduction was applied to categorical features through the binning method. Subsequently, the class imbalance dataset was addressed using the adaptive synthetic sampling data generation method. Finally, the preprocessed, refined, and oversampled feature set was divided into training and test sets with an 80–20 ratio, and two experiments were conducted. In Experiment 1, the binary classification was executed using four machine learning classifiers, with the extra trees classifier achieving the highest accuracy of 97.23% and an AUC of 0.9961. In Experiment 2, multiclass classification was performed, and the extra trees classifier emerged as the most effective, achieving an accuracy of 81.27% and an AUC of 0.97. The results were evaluated based on training, testing, and total time, and a comparative analysis with state-of-the-art studies proved the robustness and significance of the applied methods in developing a timely and precision-efficient solution to NIDS.Keywords
Cite This Article
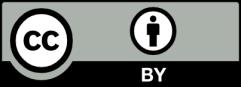