Open Access
ARTICLE
MCIF-Transformer Mask RCNN: Multi-Branch Cross-Scale Interactive Feature Fusion Transformer Model for PET/CT Lung Tumor Instance Segmentation
1 School of Medical Information & Engineering, Ningxia Medical University, Yinchuan, 750004, China
2 School of Computer Science and Engineering, North Minzu University, Yinchuan, 750021, China
3 Key Laboratory of Image and Graphics Intelligent Processing of State Ethnic Affairs Commission, North Minzu University, Yinchuan, 750021, China
* Corresponding Author: Huiling Lu. Email:
(This article belongs to the Special Issue: Deep Learning in Computer-Aided Diagnosis Based on Medical Image)
Computers, Materials & Continua 2024, 79(3), 4371-4393. https://doi.org/10.32604/cmc.2024.047827
Received 19 November 2023; Accepted 19 April 2024; Issue published 20 June 2024
Abstract
The precise detection and segmentation of tumor lesions are very important for lung cancer computer-aided diagnosis. However, in PET/CT (Positron Emission Tomography/Computed Tomography) lung images, the lesion shapes are complex, the edges are blurred, and the sample numbers are unbalanced. To solve these problems, this paper proposes a Multi-branch Cross-scale Interactive Feature fusion Transformer model (MCIF-Transformer Mask RCNN) for PET/CT lung tumor instance segmentation, The main innovative works of this paper are as follows: Firstly, the ResNet-Transformer backbone network is used to extract global feature and local feature in lung images. The pixel dependence relationship is established in local and non-local fields to improve the model perception ability. Secondly, the Cross-scale Interactive Feature Enhancement auxiliary network is designed to provide the shallow features to the deep features, and the cross-scale interactive feature enhancement module (CIFEM) is used to enhance the attention ability of the fine-grained features. Thirdly, the Cross-scale Interactive Feature fusion FPN network (CIF-FPN) is constructed to realize bidirectional interactive fusion between deep features and shallow features, and the low-level features are enhanced in deep semantic features. Finally, 4 ablation experiments, 3 comparison experiments of detection, 3 comparison experiments of segmentation and 6 comparison experiments with two-stage and single-stage instance segmentation networks are done on PET/CT lung medical image datasets. The results showed that APdet, APseg, ARdet and ARseg indexes are improved by 5.5%, 5.15%, 3.11% and 6.79% compared with Mask RCNN (resnet50). Based on the above research, the precise detection and segmentation of the lesion region are realized in this paper. This method has positive significance for the detection of lung tumors.Keywords
Cite This Article
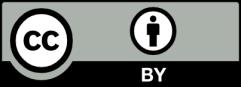
This work is licensed under a Creative Commons Attribution 4.0 International License , which permits unrestricted use, distribution, and reproduction in any medium, provided the original work is properly cited.