Open Access
ARTICLE
A Combination Prediction Model for Short Term Travel Demand of Urban Taxi
1 School of Traffic and Transportation, Beijing Jiaotong University, Beijing, 100044, China
2 Transport Planning and Research Institute, Ministry of Transport, Beijing, 100028, China
* Corresponding Author: Mingyuan Li. Email:
(This article belongs to the Special Issue: The Next-generation Deep Learning Approaches to Emerging Real-world Applications)
Computers, Materials & Continua 2024, 79(3), 3877-3896. https://doi.org/10.32604/cmc.2024.047765
Received 16 November 2023; Accepted 18 January 2024; Issue published 20 June 2024
Abstract
This study proposes a prediction model considering external weather and holiday factors to address the issue of accurately predicting urban taxi travel demand caused by complex data and numerous influencing factors. The model integrates the Complete Ensemble Empirical Mode Decomposition with Adaptive Noise (CEEMDAN) and Convolutional Long Short Term Memory Neural Network (ConvLSTM) to predict short-term taxi travel demand. The CEEMDAN decomposition method effectively decomposes time series data into a set of modal components, capturing sequence characteristics at different time scales and frequencies. Based on the sample entropy value of components, secondary processing of more complex sequence components after decomposition is employed to reduce the cumulative prediction error of component sequences and improve prediction efficiency. On this basis, considering the correlation between the spatiotemporal trends of short-term taxi traffic, a ConvLSTM neural network model with Long Short Term Memory (LSTM) time series processing ability and Convolutional Neural Networks (CNN) spatial feature processing ability is constructed to predict the travel demand for urban taxis. The combined prediction model is tested on a taxi travel demand dataset in a certain area of Beijing. The results show that the CEEMDAN-ConvLSTM prediction model outperforms the LSTM, Autoregressive Integrated Moving Average model (ARIMA), CNN, and ConvLSTM benchmark models in terms of Symmetric Mean Absolute Percentage Error (SMAPE), Root Mean Square Error (RMSE), Mean Absolute Error (MAE), and R2 metrics. Notably, the SMAPE metric exhibits a remarkable decline of 21.03% with the utilization of our proposed model. These results confirm that our study provides a highly accurate and valid model for taxi travel demand forecasting.Keywords
Cite This Article
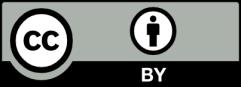