Open Access
REVIEW
A Review of NILM Applications with Machine Learning Approaches
School of Electronic and Information Engineering, Nanjing University of Information Science and Technology, Nanjing, 210044, China
* Corresponding Author: Maheesha Dhashantha Silva. Email:
Computers, Materials & Continua 2024, 79(2), 2971-2989. https://doi.org/10.32604/cmc.2024.051289
Received 01 March 2024; Accepted 11 April 2024; Issue published 15 May 2024
Abstract
In recent years, Non-Intrusive Load Monitoring (NILM) has become an emerging approach that provides affordable energy management solutions using aggregated load obtained from a single smart meter in the power grid. Furthermore, by integrating Machine Learning (ML), NILM can efficiently use electrical energy and offer less of a burden for the energy monitoring process. However, conducted research works have limitations for real-time implementation due to the practical issues. This paper aims to identify the contribution of ML approaches to developing a reliable Energy Management (EM) solution with NILM. Firstly, phases of the NILM are discussed, along with the research works that have been conducted in the domain. Secondly, the contribution of machine learning approaches in three aspects is discussed: Supervised learning, unsupervised learning, and hybrid modeling. It highlights the limitations in the applicability of ML approaches in the field. Then, the challenges in the real-time implementation are concerned with six use cases: Difficulty in recognizing multiple loads at a given time, cost of running the NILM system, lack of universal framework for appliance detection, anomaly detection and new appliance identification, and complexity of the electricity loads and real-time demand side management. Furthermore, options for selecting an approach for an efficient NILM framework are suggested. Finally, suggestions are provided for future research directions.Keywords
Cite This Article
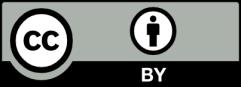
This work is licensed under a Creative Commons Attribution 4.0 International License , which permits unrestricted use, distribution, and reproduction in any medium, provided the original work is properly cited.