Open Access
ARTICLE
A Novel Approach to Energy Optimization: Efficient Path Selection in Wireless Sensor Networks with Hybrid ANN
1 Department of Electrical and Computer Engineering, International Islamic University, Islamabad, 44000, Pakistan
2 School of Information Science and Engineering, NingboTech University, Ningbo, 315100, China
3 Software Engineering Department, College of Computer and Information Sciences, King Saud University, Riyadh, 11495, Saudi Arabia
4 Computer Engineering Department, College of Computer and Information Sciences, King Saud University, Riyadh, 11495, Saudi Arabia
* Corresponding Author: Muhammad Salman Qamar. Email:
(This article belongs to the Special Issue: Advanced Artificial Intelligence and Machine Learning Frameworks for Signal and Image Processing Applications)
Computers, Materials & Continua 2024, 79(2), 2945-2970. https://doi.org/10.32604/cmc.2024.050168
Received 29 January 2024; Accepted 07 April 2024; Issue published 15 May 2024
Abstract
In pursuit of enhancing the Wireless Sensor Networks (WSNs) energy efficiency and operational lifespan, this paper delves into the domain of energy-efficient routing protocols. In WSNs, the limited energy resources of Sensor Nodes (SNs) are a big challenge for ensuring their efficient and reliable operation. WSN data gathering involves the utilization of a mobile sink (MS) to mitigate the energy consumption problem through periodic network traversal. The mobile sink (MS) strategy minimizes energy consumption and latency by visiting the fewest nodes or pre-determined locations called rendezvous points (RPs) instead of all cluster heads (CHs). CHs subsequently transmit packets to neighboring RPs. The unique determination of this study is the shortest path to reach RPs. As the mobile sink (MS) concept has emerged as a promising solution to the energy consumption problem in WSNs, caused by multi-hop data collection with static sinks. In this study, we proposed two novel hybrid algorithms, namely” Reduced k-means based on Artificial Neural Network “(RkM-ANN) and “Delay Bound Reduced k-means with ANN” (DBRkM-ANN) for designing a fast, efficient, and most proficient MS path depending upon rendezvous points (RPs). The first algorithm optimizes the MS’s latency, while the second considers the designing of delay-bound paths, also defined as the number of paths with delay over bound for the MS. Both methods use a weight function and k-means clustering to choose RPs in a way that maximizes efficiency and guarantees network-wide coverage. In addition, a method of using MS scheduling for efficient data collection is provided. Extensive simulations and comparisons to several existing algorithms have shown the effectiveness of the suggested methodologies over a wide range of performance indicators.Keywords
Cite This Article
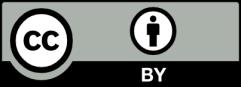
This work is licensed under a Creative Commons Attribution 4.0 International License , which permits unrestricted use, distribution, and reproduction in any medium, provided the original work is properly cited.