Open Access
ARTICLE
Optimizing Optical Fiber Faults Detection: A Comparative Analysis of Advanced Machine Learning Approaches
1 School of Electronics Engineering, Beijing University of Posts and Telecommunications, Beijing, 100876, China
2 State Key Laboratory of Integrated Services Networks, Xidian University, Xi’an, 710071, China
3 State Key Laboratory of Networking and Switching Technology, Beijing University of Posts and Telecommunications, Beijing, 100876, China
* Corresponding Author: Yuanxiang Chen. Email:
(This article belongs to the Special Issue: Advanced Artificial Intelligence and Machine Learning Frameworks for Signal and Image Processing Applications)
Computers, Materials & Continua 2024, 79(2), 2697-2721. https://doi.org/10.32604/cmc.2024.049607
Received 12 January 2024; Accepted 28 March 2024; Issue published 15 May 2024
Abstract
Efficient optical network management poses significant importance in backhaul and access network communication for preventing service disruptions and ensuring Quality of Service (QoS) satisfaction. The emerging faults in optical networks introduce challenges that can jeopardize the network with a variety of faults. The existing literature witnessed various partial or inadequate solutions. On the other hand, Machine Learning (ML) has revolutionized as a promising technique for fault detection and prevention. Unlike traditional fault management systems, this research has three-fold contributions. First, this research leverages the ML and Deep Learning (DL) multi-classification system and evaluates their accuracy in detecting six distinct fault types, including fiber cut, fiber eavesdropping, splicing, bad connector, bending, and PC connector. Secondly, this paper assesses the classification delay of each classification algorithm. Finally, this work proposes a fiber optics fault prevention algorithm that determines to mitigate the faults accordingly. This work utilized a publicly available fiber optics dataset named OTDR_Data and applied different ML classifiers, such as Gaussian Naive Bayes (GNB), Logistic Regression (LR), Support Vector Machine (SVM), K-Nearest Neighbor (KNN), Random Forest (RF), and Decision Tree (DT). Moreover, Ensemble Learning (EL) techniques are applied to evaluate the accuracy of various classifiers. In addition, this work evaluated the performance of DL-based Convolutional Neural Network and Long-Short Term Memory (CNN-LSTM) hybrid classifier. The findings reveal that the CNN-LSTM hybrid technique achieved the highest accuracy of 99% with a delay of 360 s. On the other hand, EL techniques improved the accuracy in detecting fiber optic faults. Thus, this research comprehensively assesses accuracy and delay metrics for various classifiers and proposes the most efficient attack detection system in fiber optics.Keywords
Cite This Article
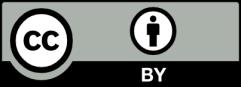