Open Access
ARTICLE
Low-Brightness Object Recognition Based on Deep Learning
Department of Information and Telecommunications Engineering, Ming Chuan University, Taoyuan City, 333, Taiwan
* Corresponding Author: Shu-Yin Chiang. Email:
(This article belongs to the Special Issue: Advanced Machine Learning and Optimization for Practical Solutions in Complex Real-world Systems)
Computers, Materials & Continua 2024, 79(2), 1757-1773. https://doi.org/10.32604/cmc.2024.049477
Received 09 January 2024; Accepted 22 March 2024; Issue published 15 May 2024
Abstract
This research focuses on addressing the challenges associated with image detection in low-light environments, particularly by applying artificial intelligence techniques to machine vision and object recognition systems. The primary goal is to tackle issues related to recognizing objects with low brightness levels. In this study, the Intel RealSense Lidar Camera L515 is used to simultaneously capture color information and 16-bit depth information images. The detection scenarios are categorized into normal brightness and low brightness situations. When the system determines a normal brightness environment, normal brightness images are recognized using deep learning methods. In low-brightness situations, three methods are proposed for recognition. The first method is the Segmentation with Depth image (SD) method which involves segmenting the depth image, creating a mask from the segmented depth image, mapping the obtained mask onto the true color (RGB) image to obtain a background-reduced RGB image, and recognizing the segmented image. The second method is the HDV method (hue, depth, value) which combines RGB images converted to HSV images (hue, saturation, value) with depth images D to form HDV images for recognition. The third method is the HSD (hue, saturation, depth) method which similarly combines RGB images converted to HSV images with depth images D to form HSD images for recognition. In experimental results, in normal brightness environments, the average recognition rate obtained using image recognition methods is 91%. For low-brightness environments, using the SD method with original images for training and segmented images for recognition achieves an average recognition rate of over 82%. The HDV method achieves an average recognition rate of over 70%, while the HSD method achieves an average recognition rate of over 84%. The HSD method allows for a quick and convenient low-light object recognition system. This research outcome can be applied to nighttime surveillance systems or nighttime road safety systems.Keywords
Cite This Article
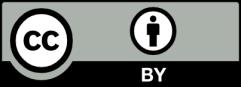
This work is licensed under a Creative Commons Attribution 4.0 International License , which permits unrestricted use, distribution, and reproduction in any medium, provided the original work is properly cited.