Open Access
ARTICLE
Hyperspectral Image Based Interpretable Feature Clustering Algorithm
1 School of Information Engineering, Yulin University, Yulin, 719000, China
2 Key Laboratory of Spectral Imaging Technology CAS, Xi’an Institute of Optics and Precision Mechanics, Chinese Academy of Sciences, Xi’an, 710119, China
* Corresponding Author: Yaming Kang. Email:
(This article belongs to the Special Issue: Advances and Applications in Signal, Image and Video Processing)
Computers, Materials & Continua 2024, 79(2), 2151-2168. https://doi.org/10.32604/cmc.2024.049360
Received 04 January 2024; Accepted 18 March 2024; Issue published 15 May 2024
Abstract
Hyperspectral imagery encompasses spectral and spatial dimensions, reflecting the material properties of objects. Its application proves crucial in search and rescue, concealed target identification, and crop growth analysis. Clustering is an important method of hyperspectral analysis. The vast data volume of hyperspectral imagery, coupled with redundant information, poses significant challenges in swiftly and accurately extracting features for subsequent analysis. The current hyperspectral feature clustering methods, which are mostly studied from space or spectrum, do not have strong interpretability, resulting in poor comprehensibility of the algorithm. So, this research introduces a feature clustering algorithm for hyperspectral imagery from an interpretability perspective. It commences with a simulated perception process, proposing an interpretable band selection algorithm to reduce data dimensions. Following this, a multi-dimensional clustering algorithm, rooted in fuzzy and kernel clustering, is developed to highlight intra-class similarities and inter-class differences. An optimized P system is then introduced to enhance computational efficiency. This system coordinates all cells within a mapping space to compute optimal cluster centers, facilitating parallel computation. This approach diminishes sensitivity to initial cluster centers and augments global search capabilities, thus preventing entrapment in local minima and enhancing clustering performance. Experiments conducted on 300 datasets, comprising both real and simulated data. The results show that the average accuracy (ACC) of the proposed algorithm is 0.86 and the combination measure (CM) is 0.81.Keywords
Cite This Article
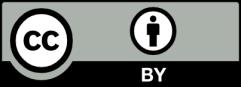
This work is licensed under a Creative Commons Attribution 4.0 International License , which permits unrestricted use, distribution, and reproduction in any medium, provided the original work is properly cited.