Open Access
ARTICLE
Predicting Age and Gender in Author Profiling: A Multi-Feature Exploration
1 Department of Computer Science, City University of Science and Information Technology, Peshawar, 25000, Pakistan
2 EIAS: Data Science and Blockchain Laboratory, College of Computer and Information Sciences, Prince Sultan University, Riyadh, 11586, Saudi Arabia
3 School of Computer Science and Technology, Guangdong University of Technology, Guangzhou, 510006, China
* Corresponding Authors: Muhammad Arshad. Email: ; Sadique Ahmad. Email:
(This article belongs to the Special Issue: Unveiling the Role of AIGC, Large Models, and Human - Centric Insights in Digital Defense)
Computers, Materials & Continua 2024, 79(2), 3333-3353. https://doi.org/10.32604/cmc.2024.049254
Received 01 January 2024; Accepted 09 March 2024; Issue published 15 May 2024
Abstract
Author Profiling (AP) is a subsection of digital forensics that focuses on the detection of the author’s personal information, such as age, gender, occupation, and education, based on various linguistic features, e.g., stylistic, semantic, and syntactic. The importance of AP lies in various fields, including forensics, security, medicine, and marketing. In previous studies, many works have been done using different languages, e.g., English, Arabic, French, etc. However, the research on Roman Urdu is not up to the mark. Hence, this study focuses on detecting the author’s age and gender based on Roman Urdu text messages. The dataset used in this study is Fire’18-MaponSMS. This study proposed an ensemble model based on AdaBoostM1 and Random Forest (AMBRF) for AP using multiple linguistic features that are stylistic, character-based, word-based, and sentence-based. The proposed model is contrasted with several of the well-known models from the literature, including J48-Decision Tree (J48), Naïve Bays (NB), K Nearest Neighbor (KNN), and Composite Hypercube on Random Projection (CHIRP), NB-Updatable, RF, and AdaboostM1. The overall outcome shows the better performance of the proposed AdaboostM1 with Random Forest (ABMRF) with an accuracy of 54.2857% for age prediction and 71.1429% for gender prediction calculated on stylistic features. Regarding word-based features, age and gender were considered in 50.5714% and 60%, respectively. On the other hand, KNN and CHIRP show the weakest performance using all the linguistic features for age and gender prediction.Keywords
Cite This Article
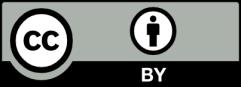
This work is licensed under a Creative Commons Attribution 4.0 International License , which permits unrestricted use, distribution, and reproduction in any medium, provided the original work is properly cited.