Open Access
ARTICLE
Posture Detection of Heart Disease Using Multi-Head Attention Vision Hybrid (MHAVH) Model
1 School of Computer Science and Engineering, Central South University, Changsha, 410003, China
2 Department of Quantitative analysis, College of Business Administration, King Saud University, P.O. Box 71115, Riyadh, 11587, Saudi Arabia
3 Electrical and Computer Engineering, University of Victoria, Victoria, V9A1B8, Canada
* Corresponding Author: Zuping Zhang. Email:
Computers, Materials & Continua 2024, 79(2), 2673-2696. https://doi.org/10.32604/cmc.2024.049186
Received 29 December 2023; Accepted 27 March 2024; Issue published 15 May 2024
Abstract
Cardiovascular disease is the leading cause of death globally. This disease causes loss of heart muscles and is also responsible for the death of heart cells, sometimes damaging their functionality. A person’s life may depend on receiving timely assistance as soon as possible. Thus, minimizing the death ratio can be achieved by early detection of heart attack (HA) symptoms. In the United States alone, an estimated 610,000 people die from heart attacks each year, accounting for one in every four fatalities. However, by identifying and reporting heart attack symptoms early on, it is possible to reduce damage and save many lives significantly. Our objective is to devise an algorithm aimed at helping individuals, particularly elderly individuals living independently, to safeguard their lives. To address these challenges, we employ deep learning techniques. We have utilized a vision transformer (ViT) to address this problem. However, it has a significant overhead cost due to its memory consumption and computational complexity because of scaling dot-product attention. Also, since transformer performance typically relies on large-scale or adequate data, adapting ViT for smaller datasets is more challenging. In response, we propose a three-in-one steam model, the Multi-Head Attention Vision Hybrid (MHAVH). This model integrates a real-time posture recognition framework to identify chest pain postures indicative of heart attacks using transfer learning techniques, such as ResNet-50 and VGG-16, renowned for their robust feature extraction capabilities. By incorporating multiple heads into the vision transformer to generate additional metrics and enhance heart-detection capabilities, we leverage a 2019 posture-based dataset comprising RGB images, a novel creation by the author that marks the first dataset tailored for posture-based heart attack detection. Given the limited online data availability, we segmented this dataset into gender categories (male and female) and conducted testing on both segmented and original datasets. The training accuracy of our model reached an impressive 99.77%. Upon testing, the accuracy for male and female datasets was recorded at 92.87% and 75.47%, respectively. The combined dataset accuracy is 93.96%, showcasing a commendable performance overall. Our proposed approach demonstrates versatility in accommodating small and large datasets, offering promising prospects for real-world applications.Keywords
Cite This Article
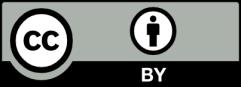