Open Access
ARTICLE
Attribute Reduction of Hybrid Decision Information Systems Based on Fuzzy Conditional Information Entropy
1 School of Big Data and Artificial Intelligence, Chizhou University, Chizhou, 247000, China
2 Anhui Education Big Data Intelligent Perception and Application Engineering Research Center, Chizhou, 247000, China
* Corresponding Author: Qinli Zhang. Email:
Computers, Materials & Continua 2024, 79(2), 2063-2083. https://doi.org/10.32604/cmc.2024.049147
Received 28 December 2023; Accepted 13 March 2024; Issue published 15 May 2024
Abstract
The presence of numerous uncertainties in hybrid decision information systems (HDISs) renders attribute reduction a formidable task. Currently available attribute reduction algorithms, including those based on Pawlak attribute importance, Skowron discernibility matrix, and information entropy, struggle to effectively manages multiple uncertainties simultaneously in HDISs like the precise measurement of disparities between nominal attribute values, and attributes with fuzzy boundaries and abnormal values. In order to address the aforementioned issues, this paper delves into the study of attribute reduction within HDISs. First of all, a novel metric based on the decision attribute is introduced to solve the problem of accurately measuring the differences between nominal attribute values. The newly introduced distance metric has been christened the supervised distance that can effectively quantify the differences between the nominal attribute values. Then, based on the newly developed metric, a novel fuzzy relationship is defined from the perspective of “feedback on parity of attribute values to attribute sets”. This new fuzzy relationship serves as a valuable tool in addressing the challenges posed by abnormal attribute values. Furthermore, leveraging the newly introduced fuzzy relationship, the fuzzy conditional information entropy is defined as a solution to the challenges posed by fuzzy attributes. It effectively quantifies the uncertainty associated with fuzzy attribute values, thereby providing a robust framework for handling fuzzy information in hybrid information systems. Finally, an algorithm for attribute reduction utilizing the fuzzy conditional information entropy is presented. The experimental results on 12 datasets show that the average reduction rate of our algorithm reaches 84.04%, and the classification accuracy is improved by 3.91% compared to the original dataset, and by an average of 11.25% compared to the other 9 state-of-the-art reduction algorithms. The comprehensive analysis of these research results clearly indicates that our algorithm is highly effective in managing the intricate uncertainties inherent in hybrid data.Keywords
Cite This Article
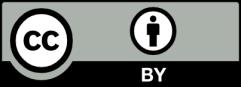