Open Access
ARTICLE
Positron Emission Tomography Lung Image Respiratory Motion Correcting with Equivariant Transformer
1 Faculty of Information Engineering and Automation, Kunming University of Science and Technology, Yunnan Key Laboratory of Artificial Intelligence, Kunming, 650500, China
2 School of Physics and Electronic Engineering, Yuxi Normal University, Yuxi, 653100, China
3 PET/CT Center, Affiliated Hospital of Kunming University of Science and Technology, First People’s Hospital of Yunnan Province, Kunming, 650031, China
* Corresponding Authors: Hui Zhou. Email: ; Bo She. Email:
(This article belongs to the Special Issue: Deep Learning in Computer-Aided Diagnosis Based on Medical Image)
Computers, Materials & Continua 2024, 79(2), 3355-3372. https://doi.org/10.32604/cmc.2024.048706
Received 15 December 2023; Accepted 21 February 2024; Issue published 15 May 2024
Abstract
In addressing the challenge of motion artifacts in Positron Emission Tomography (PET) lung scans, our study introduces the Triple Equivariant Motion Transformer (TEMT), an innovative, unsupervised, deep-learning-based framework for efficient respiratory motion correction in PET imaging. Unlike traditional techniques, which segment PET data into bins throughout a respiratory cycle and often face issues such as inefficiency and overemphasis on certain artifacts, TEMT employs Convolutional Neural Networks (CNNs) for effective feature extraction and motion decomposition.TEMT’s unique approach involves transforming motion sequences into Lie group domains to highlight fundamental motion patterns, coupled with employing competitive weighting for precise target deformation field generation. Our empirical evaluations confirm TEMT’s superior performance in handling diverse PET lung datasets compared to existing image registration networks. Experimental results demonstrate that TEMT achieved Dice indices of 91.40%, 85.41%, 79.78%, and 72.16% on simulated geometric phantom data, lung voxel phantom data, cardiopulmonary voxel phantom data, and clinical data, respectively. To facilitate further research and practical application, the TEMT framework, along with its implementation details and part of the simulation data, is made publicly accessible at https://github.com/yehaowei/temt.Keywords
Cite This Article
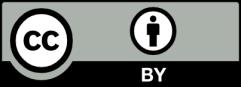
This work is licensed under a Creative Commons Attribution 4.0 International License , which permits unrestricted use, distribution, and reproduction in any medium, provided the original work is properly cited.