Open Access
ARTICLE
FusionNN: A Semantic Feature Fusion Model Based on Multimodal for Web Anomaly Detection
1 Institute of High Energy Physics, Chinese Academy of Sciences, Beijing, 100049, China
2 Spallation Neutron Source Science Center (SNSSC), Dongguan, 523803, China
3 School of Nuclear Technology, University of Chinese Academy of Sciences, Beijing, 100049, China
* Corresponding Authors: Li Wang. Email: ; Mingshan Xia. Email:
Computers, Materials & Continua 2024, 79(2), 2991-3006. https://doi.org/10.32604/cmc.2024.048637
Received 13 December 2023; Accepted 10 April 2024; Issue published 15 May 2024
Abstract
With the rapid development of the mobile communication and the Internet, the previous web anomaly detection and identification models were built relying on security experts’ empirical knowledge and attack features. Although this approach can achieve higher detection performance, it requires huge human labor and resources to maintain the feature library. In contrast, semantic feature engineering can dynamically discover new semantic features and optimize feature selection by automatically analyzing the semantic information contained in the data itself, thus reducing dependence on prior knowledge. However, current semantic features still have the problem of semantic expression singularity, as they are extracted from a single semantic mode such as word segmentation, character segmentation, or arbitrary semantic feature extraction. This paper extracts features of web requests from dual semantic granularity, and proposes a semantic feature fusion method to solve the above problems. The method first preprocesses web requests, and extracts word-level and character-level semantic features of URLs via convolutional neural network (CNN), respectively. By constructing three loss functions to reduce losses between features, labels and categories. Experiments on the HTTP CSIC 2010, Malicious URLs and HttpParams datasets verify the proposed method. Results show that compared with machine learning, deep learning methods and BERT model, the proposed method has better detection performance. And it achieved the best detection rate of 99.16% in the dataset HttpParams.Keywords
Cite This Article
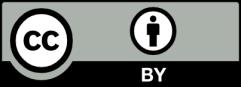
This work is licensed under a Creative Commons Attribution 4.0 International License , which permits unrestricted use, distribution, and reproduction in any medium, provided the original work is properly cited.