Open Access
ARTICLE
Fusion of Spiral Convolution-LSTM for Intrusion Detection Modeling
School of Cyberspace Security, Gansu University of Political Science and Law, Lanzhou, 73000, China
* Corresponding Author: Zhen Dong. Email:
Computers, Materials & Continua 2024, 79(2), 2315-2329. https://doi.org/10.32604/cmc.2024.048443
Received 07 December 2023; Accepted 18 March 2024; Issue published 15 May 2024
Abstract
Aiming at the problems of low accuracy and slow convergence speed of current intrusion detection models, SpiralConvolution is combined with Long Short-Term Memory Network to construct a new intrusion detection model. The dataset is first preprocessed using solo thermal encoding and normalization functions. Then the spiral convolution-Long Short-Term Memory Network model is constructed, which consists of spiral convolution, a two-layer long short-term memory network, and a classifier. It is shown through experiments that the model is characterized by high accuracy, small model computation, and fast convergence speed relative to previous deep learning models. The model uses a new neural network to achieve fast and accurate network traffic intrusion detection. The model in this paper achieves 0.9706 and 0.8432 accuracy rates on the NSL-KDD dataset and the UNSWNB-15 dataset under five classifications and ten classes, respectively.Keywords
Cite This Article
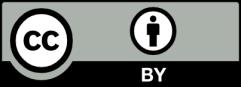
This work is licensed under a Creative Commons Attribution 4.0 International License , which permits unrestricted use, distribution, and reproduction in any medium, provided the original work is properly cited.