Open Access
ARTICLE
A Novel Approach to Breast Tumor Detection: Enhanced Speckle Reduction and Hybrid Classification in Ultrasound Imaging
1 Department of Biomedical Engineering, KIT-Kalaignarkarunanidhi Institute of Technology, Coimbatore, 641402, India
2 Graduate School, Faculty of Information Technology, Duy Tan University, Da Nang, 550000, Viet Nam
3 Department of Biomedical and Instrumentation Engineering, Avinashilingam Institute for Home Science and Higher Education for Women, Coimbatore, 641043, India
4 Universidad Michoacana de San Nicolás de Hidalgo Avenida Francisco J. Múgica S/N Ciudad Universitaria, Morelia, CP, 58030, Mexico
5 Department of Pharmacology and Toxicology, College of Pharmacy, King Saud University, Riyadh, 11451, Saudi Arabia
6 Department of Mechanical Engineering, Gujarat Technological Univeristy, Nigam Nagar, Chandkheda, Ahmedabad, Gujarat, 382424, India
* Corresponding Authors: K. Umapathi. Email: ; Hitesh Panchal. Email:
Computers, Materials & Continua 2024, 79(2), 1875-1901. https://doi.org/10.32604/cmc.2024.047961
Received 23 November 2023; Accepted 08 March 2024; Issue published 15 May 2024
Abstract
Breast cancer detection heavily relies on medical imaging, particularly ultrasound, for early diagnosis and effective treatment. This research addresses the challenges associated with computer-aided diagnosis (CAD) of breast cancer from ultrasound images. The primary challenge is accurately distinguishing between malignant and benign tumors, complicated by factors such as speckle noise, variable image quality, and the need for precise segmentation and classification. The main objective of the research paper is to develop an advanced methodology for breast ultrasound image classification, focusing on speckle noise reduction, precise segmentation, feature extraction, and machine learning-based classification. A unique approach is introduced that combines Enhanced Speckle Reduced Anisotropic Diffusion (SRAD) filters for speckle noise reduction, U-NET-based segmentation, Genetic Algorithm (GA)-based feature selection, and Random Forest and Bagging Tree classifiers, resulting in a novel and efficient model. To test and validate the hybrid model, rigorous experimentations were performed and results state that the proposed hybrid model achieved accuracy rate of 99.9%, outperforming other existing techniques, and also significantly reducing computational time. This enhanced accuracy, along with improved sensitivity and specificity, makes the proposed hybrid model a valuable addition to CAD systems in breast cancer diagnosis, ultimately enhancing diagnostic accuracy in clinical applications.Keywords
Cite This Article
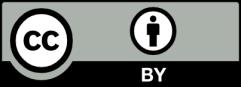
This work is licensed under a Creative Commons Attribution 4.0 International License , which permits unrestricted use, distribution, and reproduction in any medium, provided the original work is properly cited.