Open Access
ARTICLE
Graph Convolutional Networks Embedding Textual Structure Information for Relation Extraction
School of Electrical and Information Engineering, Beijing University of Civil Engineering and Architecture, Beijing, 102616, China
* Corresponding Author: Chuyuan Wei. Email:
Computers, Materials & Continua 2024, 79(2), 3299-3314. https://doi.org/10.32604/cmc.2024.047811
Received 18 November 2023; Accepted 19 February 2024; Issue published 15 May 2024
Abstract
Deep neural network-based relational extraction research has made significant progress in recent years, and it provides data support for many natural language processing downstream tasks such as building knowledge graph, sentiment analysis and question-answering systems. However, previous studies ignored much unused structural information in sentences that could enhance the performance of the relation extraction task. Moreover, most existing dependency-based models utilize self-attention to distinguish the importance of context, which hardly deals with multiple-structure information. To efficiently leverage multiple structure information, this paper proposes a dynamic structure attention mechanism model based on textual structure information, which deeply integrates word embedding, named entity recognition labels, part of speech, dependency tree and dependency type into a graph convolutional network. Specifically, our model extracts text features of different structures from the input sentence. Textual Structure information Graph Convolutional Networks employs the dynamic structure attention mechanism to learn multi-structure attention, effectively distinguishing important contextual features in various structural information. In addition, multi-structure weights are carefully designed as a merging mechanism in the different structure attention to dynamically adjust the final attention. This paper combines these features and trains a graph convolutional network for relation extraction. We experiment on supervised relation extraction datasets including SemEval 2010 Task 8, TACRED, TACREV, and Re-TACED, the result significantly outperforms the previous.Keywords
Cite This Article
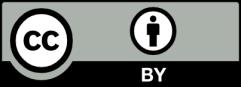
This work is licensed under a Creative Commons Attribution 4.0 International License , which permits unrestricted use, distribution, and reproduction in any medium, provided the original work is properly cited.