Open Access
ARTICLE
Cluster Detection Method of Endogenous Security Abnormal Attack Behavior in Air Traffic Control Network
1 College of Computer Science, Sichuan University, Chengdu, 610065, China
2 Equipment Management and Unmanned Aerial Vehicle College of Air Force Engineering University, Air Force Engineering University, Xi’an, 710051, China
* Corresponding Author: Jianwei Zhang. Email:
(This article belongs to the Special Issue: AI and Data Security for the Industrial Internet)
Computers, Materials & Continua 2024, 79(2), 2523-2546. https://doi.org/10.32604/cmc.2024.047543
Received 08 November 2023; Accepted 13 March 2024; Issue published 15 May 2024
Abstract
In order to enhance the accuracy of Air Traffic Control (ATC) cybersecurity attack detection, in this paper, a new clustering detection method is designed for air traffic control network security attacks. The feature set for ATC cybersecurity attacks is constructed by setting the feature states, adding recursive features, and determining the feature criticality. The expected information gain and entropy of the feature data are computed to determine the information gain of the feature data and reduce the interference of similar feature data. An autoencoder is introduced into the AI (artificial intelligence) algorithm to encode and decode the characteristics of ATC network security attack behavior to reduce the dimensionality of the ATC network security attack behavior data. Based on the above processing, an unsupervised learning algorithm for clustering detection of ATC network security attacks is designed. First, determine the distance between the clustering clusters of ATC network security attack behavior characteristics, calculate the clustering threshold, and construct the initial clustering center. Then, the new average value of all feature objects in each cluster is recalculated as the new cluster center. Second, it traverses all objects in a cluster of ATC network security attack behavior feature data. Finally, the cluster detection of ATC network security attack behavior is completed by the computation of objective functions. The experiment took three groups of experimental attack behavior data sets as the test object, and took the detection rate, false detection rate and recall rate as the test indicators, and selected three similar methods for comparative test. The experimental results show that the detection rate of this method is about 98%, the false positive rate is below 1%, and the recall rate is above 97%. Research shows that this method can improve the detection performance of security attacks in air traffic control network.Keywords
Cite This Article
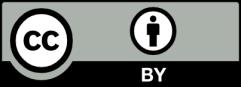