Open Access
ARTICLE
Faster Region Convolutional Neural Network (FRCNN) Based Facial Emotion Recognition
1 Department of Electronics and Communication Engineering, Karunya Institute of Technology and Sciences, Coimbatore, 641114, India
2 College of Engineering and Technology, American University of the Middle East, Egaila, 54200, Kuwait
3 Department of Civil Engineering, Karunya Institute of Technology and Sciences, Coimbatore, 641114, India
* Corresponding Author: A. Diana Andrushia. Email:
Computers, Materials & Continua 2024, 79(2), 2427-2448. https://doi.org/10.32604/cmc.2024.047326
Received 02 November 2023; Accepted 18 March 2024; Issue published 15 May 2024
Abstract
Facial emotion recognition (FER) has become a focal point of research due to its widespread applications, ranging from human-computer interaction to affective computing. While traditional FER techniques have relied on handcrafted features and classification models trained on image or video datasets, recent strides in artificial intelligence and deep learning (DL) have ushered in more sophisticated approaches. The research aims to develop a FER system using a Faster Region Convolutional Neural Network (FRCNN) and design a specialized FRCNN architecture tailored for facial emotion recognition, leveraging its ability to capture spatial hierarchies within localized regions of facial features. The proposed work enhances the accuracy and efficiency of facial emotion recognition. The proposed work comprises two major key components: Inception V3-based feature extraction and FRCNN-based emotion categorization. Extensive experimentation on Kaggle datasets validates the effectiveness of the proposed strategy, showcasing the FRCNN approach’s resilience and accuracy in identifying and categorizing facial expressions. The model’s overall performance metrics are compelling, with an accuracy of 98.4%, precision of 97.2%, and recall of 96.31%. This work introduces a perceptive deep learning-based FER method, contributing to the evolving landscape of emotion recognition technologies. The high accuracy and resilience demonstrated by the FRCNN approach underscore its potential for real-world applications. This research advances the field of FER and presents a compelling case for the practicality and efficacy of deep learning models in automating the understanding of facial emotions.Keywords
Cite This Article
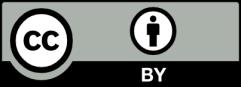
This work is licensed under a Creative Commons Attribution 4.0 International License , which permits unrestricted use, distribution, and reproduction in any medium, provided the original work is properly cited.