Open Access
REVIEW
Survey of Indoor Localization Based on Deep Learning
1 Centre for Wireless Technology, Faculty of Engineering, Multimedia University, Cyberjaya, 63100, Malaysia
2 Communication Systems & Networks Research Lab, Malaysia-Japan International Institute of Technology, Universiti Teknologi Malaysia, Kuala Lumpur, 54100, Malaysia
3 Department of Electrical Engineering (DEE), International Islamic University, Islamabad, 44000, Pakistan
4 Rohde & Schwarz (M) Sdn Bhd, Shah Alam, 40150, Malaysia
* Corresponding Author: Mardeni Roslee. Email:
Computers, Materials & Continua 2024, 79(2), 3261-3298. https://doi.org/10.32604/cmc.2024.044890
Received 11 August 2023; Accepted 14 December 2023; Issue published 15 May 2024
Abstract
This study comprehensively examines the current state of deep learning (DL) usage in indoor positioning. It emphasizes the significance and efficiency of convolutional neural networks (CNNs) and recurrent neural networks (RNNs). Unlike prior studies focused on single sensor modalities like Wi-Fi or Bluetooth, this research explores the integration of multiple sensor modalities (e.g., Wi-Fi, Bluetooth, Ultra-Wideband, ZigBee) to expand indoor localization methods, particularly in obstructed environments. It addresses the challenge of precise object localization, introducing a novel hybrid DL approach using received signal information (RSI), Received Signal Strength (RSS), and Channel State Information (CSI) data to enhance accuracy and stability. Moreover, the study introduces a device-free indoor localization algorithm, offering a significant advancement with potential object or individual tracking applications. It recognizes the increasing importance of indoor positioning for location-based services. It anticipates future developments while acknowledging challenges such as multipath interference, noise, data standardization, and scarcity of labeled data. This research contributes significantly to indoor localization technology, offering adaptability, device independence, and multifaceted DL-based solutions for real-world challenges and future advancements. Thus, the proposed work addresses challenges in object localization precision and introduces a novel hybrid deep learning approach, contributing to advancing location-centric services. While deep learning-based indoor localization techniques have improved accuracy, challenges like data noise, standardization, and availability of training data persist. However, ongoing developments are expected to enhance indoor positioning systems to meet real-world demands.Keywords
Cite This Article
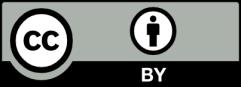
This work is licensed under a Creative Commons Attribution 4.0 International License , which permits unrestricted use, distribution, and reproduction in any medium, provided the original work is properly cited.