Open Access
ARTICLE
Complementary-Label Adversarial Domain Adaptation Fault Diagnosis Network under Time-Varying Rotational Speed and Weakly-Supervised Conditions
1 School of Data Science and Technology, North University of China, Taiyuan, 030051, China
2 School of Mechanical Engineering, North University of China, Taiyuan, 030051, China
* Corresponding Author: Siyuan Liu. Email:
(This article belongs to the Special Issue: Industrial Big Data and Artificial Intelligence-Driven Intelligent Perception, Maintenance, and Decision Optimization in Industrial Systems)
Computers, Materials & Continua 2024, 79(1), 761-777. https://doi.org/10.32604/cmc.2024.049484
Received 09 January 2024; Accepted 26 February 2024; Issue published 25 April 2024
Abstract
Recent research in cross-domain intelligence fault diagnosis of machinery still has some problems, such as relatively ideal speed conditions and sample conditions. In engineering practice, the rotational speed of the machine is often transient and time-varying, which makes the sample annotation increasingly expensive. Meanwhile, the number of samples collected from different health states is often unbalanced. To deal with the above challenges, a complementary-label (CL) adversarial domain adaptation fault diagnosis network (CLADAN) is proposed under time-varying rotational speed and weakly-supervised conditions. In the weakly supervised learning condition, machine prior information is used for sample annotation via cost-friendly complementary label learning. A diagnostic model learning strategy with discretized category probabilities is designed to avoid multi-peak distribution of prediction results. In adversarial training process, we developed virtual adversarial regularization (VAR) strategy, which further enhances the robustness of the model by adding adversarial perturbations in the target domain. Comparative experiments on two case studies validated the superior performance of the proposed method.Keywords
Cite This Article
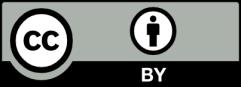
This work is licensed under a Creative Commons Attribution 4.0 International License , which permits unrestricted use, distribution, and reproduction in any medium, provided the original work is properly cited.