Open Access
ARTICLE
CrossFormer Embedding DeepLabv3+ for Remote Sensing Images Semantic Segmentation
School of Information Science and Technology, Hainan Normal University, Haikou, 571158, China
* Corresponding Author: Haihua Xing. Email:
(This article belongs to the Special Issue: Advances and Applications in Signal, Image and Video Processing)
Computers, Materials & Continua 2024, 79(1), 1353-1375. https://doi.org/10.32604/cmc.2024.049187
Received 29 December 2023; Accepted 14 March 2024; Issue published 25 April 2024
Abstract
High-resolution remote sensing image segmentation is a challenging task. In urban remote sensing, the presence of occlusions and shadows often results in blurred or invisible object boundaries, thereby increasing the difficulty of segmentation. In this paper, an improved network with a cross-region self-attention mechanism for multi-scale features based on DeepLabv3+ is designed to address the difficulties of small object segmentation and blurred target edge segmentation. First, we use CrossFormer as the backbone feature extraction network to achieve the interaction between large- and small-scale features, and establish self-attention associations between features at both large and small scales to capture global contextual feature information. Next, an improved atrous spatial pyramid pooling module is introduced to establish multi-scale feature maps with large- and small-scale feature associations, and attention vectors are added in the channel direction to enable adaptive adjustment of multi-scale channel features. The proposed network model is validated using the Potsdam and Vaihingen datasets. The experimental results show that, compared with existing techniques, the network model designed in this paper can extract and fuse multi-scale information, more clearly extract edge information and small-scale information, and segment boundaries more smoothly. Experimental results on public datasets demonstrate the superiority of our method compared with several state-of-the-art networks.Keywords
Cite This Article
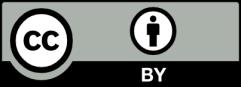
This work is licensed under a Creative Commons Attribution 4.0 International License , which permits unrestricted use, distribution, and reproduction in any medium, provided the original work is properly cited.