Open Access
ARTICLE
Efficient Unsupervised Image Stitching Using Attention Mechanism with Deep Homography Estimation
School of Artificial Intelligence, Henan University, Zhengzhou, 450000, China
* Corresponding Author: Chunbin Qin. Email:
(This article belongs to the Special Issue: Advances and Applications in Signal, Image and Video Processing)
Computers, Materials & Continua 2024, 79(1), 1319-1334. https://doi.org/10.32604/cmc.2024.048850
Received 20 December 2023; Accepted 13 March 2024; Issue published 25 April 2024
Abstract
Traditional feature-based image stitching techniques often encounter obstacles when dealing with images lacking unique attributes or suffering from quality degradation. The scarcity of annotated datasets in real-life scenes severely undermines the reliability of supervised learning methods in image stitching. Furthermore, existing deep learning architectures designed for image stitching are often too bulky to be deployed on mobile and peripheral computing devices. To address these challenges, this study proposes a novel unsupervised image stitching method based on the YOLOv8 (You Only Look Once version 8) framework that introduces deep homography networks and attention mechanisms. The methodology is partitioned into three distinct stages. The initial stage combines the attention mechanism with a pooling pyramid model to enhance the detection and recognition of compact objects in images, the task of the deep homography networks module is to estimate the global homography of the input images considering multiple viewpoints. The second stage involves preliminary stitching of the masks generated in the initial stage and further enhancement through weighted computation to eliminate common stitching artifacts. The final stage is characterized by adaptive reconstruction and careful refinement of the initial stitching results. Comprehensive experiments across multiple datasets are executed to meticulously assess the proposed model. Our method’s Peak Signal-to-Noise Ratio (PSNR) and Structure Similarity Index Measure (SSIM) improved by 10.6% and 6%. These experimental results confirm the efficacy and utility of the presented model in this paper.Keywords
Cite This Article
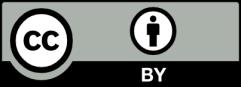
This work is licensed under a Creative Commons Attribution 4.0 International License , which permits unrestricted use, distribution, and reproduction in any medium, provided the original work is properly cited.