Open Access
ARTICLE
Safety-Constrained Multi-Agent Reinforcement Learning for Power Quality Control in Distributed Renewable Energy Networks
Department of Computer Engineering, Chonnam National University, Yeosu, 59626, South Korea
* Corresponding Author: Chang Cyoon Lim. Email:
(This article belongs to the Special Issue: Edge Computing in Advancing the Capabilities of Smart Cities)
Computers, Materials & Continua 2024, 79(1), 449-471. https://doi.org/10.32604/cmc.2024.048771
Received 18 December 2023; Accepted 19 February 2024; Issue published 25 April 2024
Abstract
This paper examines the difficulties of managing distributed power systems, notably due to the increasing use of renewable energy sources, and focuses on voltage control challenges exacerbated by their variable nature in modern power grids. To tackle the unique challenges of voltage control in distributed renewable energy networks, researchers are increasingly turning towards multi-agent reinforcement learning (MARL). However, MARL raises safety concerns due to the unpredictability in agent actions during their exploration phase. This unpredictability can lead to unsafe control measures. To mitigate these safety concerns in MARL-based voltage control, our study introduces a novel approach: Safety-Constrained Multi-Agent Reinforcement Learning (SC-MARL). This approach incorporates a specialized safety constraint module specifically designed for voltage control within the MARL framework. This module ensures that the MARL agents carry out voltage control actions safely. The experiments demonstrate that, in the 33-buses, 141-buses, and 322-buses power systems, employing SC-MARL for voltage control resulted in a reduction of the Voltage Out of Control Rate (%V.out) from 0.43, 0.24, and 2.95 to 0, 0.01, and 0.03, respectively. Additionally, the Reactive Power Loss (Q loss) decreased from 0.095, 0.547, and 0.017 to 0.062, 0.452, and 0.016 in the corresponding systems.Keywords
Cite This Article
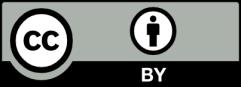
This work is licensed under a Creative Commons Attribution 4.0 International License , which permits unrestricted use, distribution, and reproduction in any medium, provided the original work is properly cited.