Open Access
ARTICLE
Sentiment Analysis of Low-Resource Language Literature Using Data Processing and Deep Learning
1 Department of IT and Computer Science, Pak-Austria Fachhochschule: Institute of Applied Sciences and Technology, Haripur, 22620, Pakistan
2 Software Competence Center Hagenberg, Softwarepark 32a, Hagenberg, 4232, Austria
3 Department of Computer Science, School of Engineering and Digital Sciences, Nazarbayev University, Astana, 010000, Kazakhstan
4 Department of Information Technology, College of Computer, Qassim University, P.O.Box 1162, Buraydah, Saudi Arabia
* Corresponding Author: Abdulrahman Aloraini. Email:
(This article belongs to the Special Issue: Advance Machine Learning for Sentiment Analysis over Various Domains and Applications)
Computers, Materials & Continua 2024, 79(1), 713-733. https://doi.org/10.32604/cmc.2024.048712
Received 16 December 2023; Accepted 19 February 2024; Issue published 25 April 2024
Abstract
Sentiment analysis, a crucial task in discerning emotional tones within the text, plays a pivotal role in understanding public opinion and user sentiment across diverse languages. While numerous scholars conduct sentiment analysis in widely spoken languages such as English, Chinese, Arabic, Roman Arabic, and more, we come to grappling with resource-poor languages like Urdu literature which becomes a challenge. Urdu is a uniquely crafted language, characterized by a script that amalgamates elements from diverse languages, including Arabic, Parsi, Pashtu, Turkish, Punjabi, Saraiki, and more. As Urdu literature, characterized by distinct character sets and linguistic features, presents an additional hurdle due to the lack of accessible datasets, rendering sentiment analysis a formidable undertaking. The limited availability of resources has fueled increased interest among researchers, prompting a deeper exploration into Urdu sentiment analysis. This research is dedicated to Urdu language sentiment analysis, employing sophisticated deep learning models on an extensive dataset categorized into five labels: Positive, Negative, Neutral, Mixed, and Ambiguous. The primary objective is to discern sentiments and emotions within the Urdu language, despite the absence of well-curated datasets. To tackle this challenge, the initial step involves the creation of a comprehensive Urdu dataset by aggregating data from various sources such as newspapers, articles, and social media comments. Subsequent to this data collection, a thorough process of cleaning and preprocessing is implemented to ensure the quality of the data. The study leverages two well-known deep learning models, namely Convolutional Neural Networks (CNN) and Recurrent Neural Networks (RNN), for both training and evaluating sentiment analysis performance. Additionally, the study explores hyperparameter tuning to optimize the models’ efficacy. Evaluation metrics such as precision, recall, and the F1-score are employed to assess the effectiveness of the models. The research findings reveal that RNN surpasses CNN in Urdu sentiment analysis, gaining a significantly higher accuracy rate of 91%. This result accentuates the exceptional performance of RNN, solidifying its status as a compelling option for conducting sentiment analysis tasks in the Urdu language.Keywords
Cite This Article
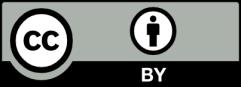