Open Access
ARTICLE
Anomaly Detection Algorithm of Power System Based on Graph Structure and Anomaly Attention
Zhaoqing Power Supply Bureau of Guangdong Power Grid Co., Ltd., Zhaoqing, 526060, China
* Corresponding Author: Yifan Gao. Email:
(This article belongs to the Special Issue: AI and Data Security for the Industrial Internet)
Computers, Materials & Continua 2024, 79(1), 493-507. https://doi.org/10.32604/cmc.2024.048615
Received 13 December 2023; Accepted 11 February 2024; Issue published 25 April 2024
Abstract
In this paper, we propose a novel anomaly detection method for data centers based on a combination of graph structure and abnormal attention mechanism. The method leverages the sensor monitoring data from target power substations to construct multidimensional time series. These time series are subsequently transformed into graph structures, and corresponding adjacency matrices are obtained. By incorporating the adjacency matrices and additional weights associated with the graph structure, an aggregation matrix is derived. The aggregation matrix is then fed into a pre-trained graph convolutional neural network (GCN) to extract graph structure features. Moreover, both the multidimensional time series segments and the graph structure features are inputted into a pre-trained anomaly detection model, resulting in corresponding anomaly detection results that help identify abnormal data. The anomaly detection model consists of a multi-level encoder-decoder module, wherein each level includes a transformer encoder and decoder based on correlation differences. The attention module in the encoding layer adopts an abnormal attention module with a dual-branch structure. Experimental results demonstrate that our proposed method significantly improves the accuracy and stability of anomaly detection.Keywords
Cite This Article
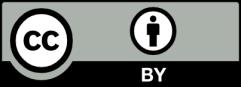
This work is licensed under a Creative Commons Attribution 4.0 International License , which permits unrestricted use, distribution, and reproduction in any medium, provided the original work is properly cited.