Open Access
ARTICLE
Weakly Supervised Network with Scribble-Supervised and Edge-Mask for Road Extraction from High-Resolution Remote Sensing Images
1 College of Artificial Intelligence, Nanjing Agricultural University, Nanjing, 210095, China
2 Innovation Academy for Microsatellites of CAS, Shanghai, 201210, China
3 Shanghai Engineering Center for Microsatellites, Shanghai, 201210, China
4 Key Laboratory of Satellite Digital Technology, Shanghai, 201210, China
* Corresponding Authors: Fen Huang. Email: ; Chengcheng Fan. Email:
Computers, Materials & Continua 2024, 79(1), 549-562. https://doi.org/10.32604/cmc.2024.048608
Received 13 December 2023; Accepted 19 February 2024; Issue published 25 April 2024
Abstract
Significant advancements have been achieved in road surface extraction based on high-resolution remote sensing image processing. Most current methods rely on fully supervised learning, which necessitates enormous human effort to label the image. Within this field, other research endeavors utilize weakly supervised methods. These approaches aim to reduce the expenses associated with annotation by leveraging sparsely annotated data, such as scribbles. This paper presents a novel technique called a weakly supervised network using scribble-supervised and edge-mask (WSSE-net). This network is a three-branch network architecture, whereby each branch is equipped with a distinct decoder module dedicated to road extraction tasks. One of the branches is dedicated to generating edge masks using edge detection algorithms and optimizing road edge details. The other two branches supervise the model’s training by employing scribble labels and spreading scribble information throughout the image. To address the historical flaw that created pseudo-labels that are not updated with network training, we use mixup to blend prediction results dynamically and continually update new pseudo-labels to steer network training. Our solution demonstrates efficient operation by simultaneously considering both edge-mask aid and dynamic pseudo-label support. The studies are conducted on three separate road datasets, which consist primarily of high-resolution remote-sensing satellite photos and drone images. The experimental findings suggest that our methodology performs better than advanced scribble-supervised approaches and specific traditional fully supervised methods.Keywords
Cite This Article
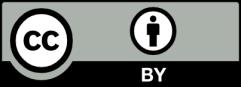
This work is licensed under a Creative Commons Attribution 4.0 International License , which permits unrestricted use, distribution, and reproduction in any medium, provided the original work is properly cited.