Open Access
ARTICLE
A Study on Enhancing Chip Detection Efficiency Using the Lightweight Van-YOLOv8 Network
School of Mechanical Engineering and Automation, Dalian Polytechnic University, Dalian, 116034, China
* Corresponding Author: Honglei Wei. Email:
(This article belongs to the Special Issue: Neural Architecture Search: Optimization, Efficiency and Application)
Computers, Materials & Continua 2024, 79(1), 531-547. https://doi.org/10.32604/cmc.2024.048510
Received 10 December 2023; Accepted 11 February 2024; Issue published 25 April 2024
Abstract
In pursuit of cost-effective manufacturing, enterprises are increasingly adopting the practice of utilizing recycled semiconductor chips. To ensure consistent chip orientation during packaging, a circular marker on the front side is employed for pin alignment following successful functional testing. However, recycled chips often exhibit substantial surface wear, and the identification of the relatively small marker proves challenging. Moreover, the complexity of generic target detection algorithms hampers seamless deployment. Addressing these issues, this paper introduces a lightweight YOLOv8s-based network tailored for detecting markings on recycled chips, termed Van-YOLOv8. Initially, to alleviate the influence of diminutive, low-resolution markings on the precision of deep learning models, we utilize an upscaling approach for enhanced resolution. This technique relies on the Super-Resolution Generative Adversarial Network with Extended Training (SRGANext) network, facilitating the reconstruction of high-fidelity images that align with input specifications. Subsequently, we replace the original YOLOv8s model’s backbone feature extraction network with the lightweight Vanilla Network (VanillaNet), simplifying the branch structure to reduce network parameters. Finally, a Hybrid Attention Mechanism (HAM) is implemented to capture essential details from input images, improving feature representation while concurrently expediting model inference speed. Experimental results demonstrate that the Van-YOLOv8 network outperforms the original YOLOv8s on a recycled chip dataset in various aspects. Significantly, it demonstrates superiority in parameter count, computational intricacy, precision in identifying targets, and speed when compared to certain prevalent algorithms in the current landscape. The proposed approach proves promising for real-time detection of recycled chips in practical factory settings.Keywords
Cite This Article
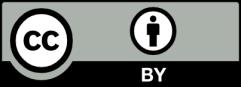