Open Access
ARTICLE
A Deep Learning Framework for Mass-Forming Chronic Pancreatitis and Pancreatic Ductal Adenocarcinoma Classification Based on Magnetic Resonance Imaging
1 Faculty of Information Engineering and Automation, Kunming University of Science and Technology, Kunming, 650504, China
2 Department of Radiology, Second Affiliated Hospital of Kunming Medical University, Kunming, 650101, China
3 School of Physics and Electronic Engineering, Yuxi Normal University, Yuxi, 653100, China
* Corresponding Authors: Jingang Hao. Email: ; Jianfeng He. Email:
(This article belongs to the Special Issue: Deep Learning in Computer-Aided Diagnosis Based on Medical Image)
Computers, Materials & Continua 2024, 79(1), 409-427. https://doi.org/10.32604/cmc.2024.048507
Received 10 December 2023; Accepted 11 February 2024; Issue published 25 April 2024
Abstract
Pancreatic diseases, including mass-forming chronic pancreatitis (MFCP) and pancreatic ductal adenocarcinoma (PDAC), present with similar imaging features, leading to diagnostic complexities. Deep Learning (DL) methods have been shown to perform well on diagnostic tasks. Existing DL pancreatic lesion diagnosis studies based on Magnetic Resonance Imaging (MRI) utilize the prior information to guide models to focus on the lesion region. However, over-reliance on prior information may ignore the background information that is helpful for diagnosis. This study verifies the diagnostic significance of the background information using a clinical dataset. Consequently, the Prior Difference Guidance Network (PDGNet) is proposed, merging decoupled lesion and background information via the Prior Normalization Fusion (PNF) strategy and the Feature Difference Guidance (FDG) module, to direct the model to concentrate on beneficial regions for diagnosis. Extensive experiments in the clinical dataset demonstrate that the proposed method achieves promising diagnosis performance: PDGNets based on conventional networks record an ACC (Accuracy) and AUC (Area Under the Curve) of 87.50% and 89.98%, marking improvements of 8.19% and 7.64% over the prior-free benchmark. Compared to lesion-focused benchmarks, the uplift is 6.14% and 6.02%. PDGNets based on advanced networks reach an ACC and AUC of 89.77% and 92.80%. The study underscores the potential of harnessing background information in medical image diagnosis, suggesting a more holistic view for future research.Keywords
Cite This Article
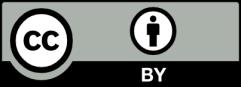