Open Access
ARTICLE
Multi-Objective Optimization Algorithm for Grouping Decision Variables Based on Extreme Point Pareto Frontier
1 College of Computer Science and Technology, Shenyang University of Chemical Technology, Shenyang, 110142, China
2 Key Laboratory of Intelligent Technology for Chemical Process Industry of Liaoning Province, Shenyang University of Chemical Technology, Shenyang, 110142, China
3 Industrial Engineering Department, College of Engineering, King Saud University, P.O. Box 800, Riyadh, 11421, Saudi Arabia
* Corresponding Author: Funan Peng. Email:
(This article belongs to the Special Issue: Recent Advances in Ensemble Framework of Meta-heuristics and Machine Learning: Methods and Applications)
Computers, Materials & Continua 2024, 79(1), 1281-1299. https://doi.org/10.32604/cmc.2024.048495
Received 09 December 2023; Accepted 04 March 2024; Issue published 25 April 2024
Abstract
The existing algorithms for solving multi-objective optimization problems fall into three main categories: Decomposition-based, dominance-based, and indicator-based. Traditional multi-objective optimization problems mainly focus on objectives, treating decision variables as a total variable to solve the problem without considering the critical role of decision variables in objective optimization. As seen, a variety of decision variable grouping algorithms have been proposed. However, these algorithms are relatively broad for the changes of most decision variables in the evolution process and are time-consuming in the process of finding the Pareto frontier. To solve these problems, a multi-objective optimization algorithm for grouping decision variables based on extreme point Pareto frontier (MOEA-DV/EPF) is proposed. This algorithm adopts a preprocessing rule to solve the Pareto optimal solution set of extreme points generated by simultaneous evolution in various target directions, obtains the basic Pareto front surface to determine the convergence effect, and analyzes the convergence and distribution effects of decision variables. In the later stages of algorithm optimization, different mutation strategies are adopted according to the nature of the decision variables to speed up the rate of evolution to obtain excellent individuals, thus enhancing the performance of the algorithm. Evaluation validation of the test functions shows that this algorithm can solve the multi-objective optimization problem more efficiently.Keywords
Cite This Article
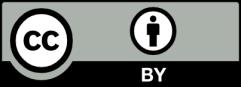
This work is licensed under a Creative Commons Attribution 4.0 International License , which permits unrestricted use, distribution, and reproduction in any medium, provided the original work is properly cited.