Open Access
ARTICLE
Mobile Crowdsourcing Task Allocation Based on Dynamic Self-Attention GANs
1 School of Computer and Control Engineering, Yantai University, Yantai, 264005, China
2 School of Computer and Information Science, Southwest University, Chongqing, 400715, China
* Corresponding Author: Qingxian Pan. Email:
(This article belongs to the Special Issue: Edge Computing in Advancing the Capabilities of Smart Cities)
Computers, Materials & Continua 2024, 79(1), 607-622. https://doi.org/10.32604/cmc.2024.048240
Received 01 December 2023; Accepted 19 February 2024; Issue published 25 April 2024
Abstract
Crowdsourcing technology is widely recognized for its effectiveness in task scheduling and resource allocation. While traditional methods for task allocation can help reduce costs and improve efficiency, they may encounter challenges when dealing with abnormal data flow nodes, leading to decreased allocation accuracy and efficiency. To address these issues, this study proposes a novel two-part invalid detection task allocation framework. In the first step, an anomaly detection model is developed using a dynamic self-attentive GAN to identify anomalous data. Compared to the baseline method, the model achieves an approximately 4% increase in the F1 value on the public dataset. In the second step of the framework, task allocation modeling is performed using a two-part graph matching method. This phase introduces a P-queue KM algorithm that implements a more efficient optimization strategy. The allocation efficiency is improved by approximately 23.83% compared to the baseline method. Empirical results confirm the effectiveness of the proposed framework in detecting abnormal data nodes, enhancing allocation precision, and achieving efficient allocation.Keywords
Cite This Article
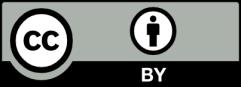
This work is licensed under a Creative Commons Attribution 4.0 International License , which permits unrestricted use, distribution, and reproduction in any medium, provided the original work is properly cited.