Open Access
ARTICLE
A Dual Discriminator Method for Generalized Zero-Shot Learning
1 School of Computer Science and Technology, Harbin University of Science and Technology, Harbin, 150006, China
2 School of Automation, Harbin University of Science and Technology, Harbin, 150006, China
* Corresponding Author: Jinjie Huang. Email:
Computers, Materials & Continua 2024, 79(1), 1599-1612. https://doi.org/10.32604/cmc.2024.048098
Received 27 November 2023; Accepted 18 March 2024; Issue published 25 April 2024
Abstract
Zero-shot learning enables the recognition of new class samples by migrating models learned from semantic features and existing sample features to things that have never been seen before. The problems of consistency of different types of features and domain shift problems are two of the critical issues in zero-shot learning. To address both of these issues, this paper proposes a new modeling structure. The traditional approach mapped semantic features and visual features into the same feature space; based on this, a dual discriminator approach is used in the proposed model. This dual discriminator approach can further enhance the consistency between semantic and visual features. At the same time, this approach can also align unseen class semantic features and training set samples, providing a portion of information about the unseen classes. In addition, a new feature fusion method is proposed in the model. This method is equivalent to adding perturbation to the seen class features, which can reduce the degree to which the classification results in the model are biased towards the seen classes. At the same time, this feature fusion method can provide part of the information of the unseen classes, improving its classification accuracy in generalized zero-shot learning and reducing domain bias. The proposed method is validated and compared with other methods on four datasets, and from the experimental results, it can be seen that the method proposed in this paper achieves promising results.Keywords
Cite This Article
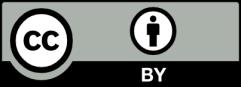
This work is licensed under a Creative Commons Attribution 4.0 International License , which permits unrestricted use, distribution, and reproduction in any medium, provided the original work is properly cited.