Open Access
ARTICLE
Securing Cloud-Encrypted Data: Detecting Ransomware-as-a-Service (RaaS) Attacks through Deep Learning Ensemble
1 School of Computer Information Sciences, University of Cumberlands, Williamsburg, Kentucky, 40769, USA
2 Computer Science Department, College of Computer Science and Information Systems, Najran University, Najran, 61441, Saudi Arabia
3 Department of Mechanical Engineering, College of Engineering, King Faisal University, Al-Ahsa, 31982, Saudi Arabia
4 Department of Electrical, Electronics and Computer Systems, College of Engineering and Technology, University of Sargodha, Sargodha, 40100, Pakistan
5 Electrical Engineering Department, College of Engineering, Najran University, Najran, 61441, Saudi Arabia
6 Department of Electrical Engineering, The University of Lahore, Lahore, 54000, Pakistan
7 Faculty of Computer and Information Systems, Islamic University of Madinah, Madinah, 42351, Saudi Arabia
8 Department of Information Systems, College of Computer and Information Sciences, Princess Nourah bint Abdulrahman University, P.O. Box 84428, Riyadh, 11671, Saudi Arabia
* Corresponding Author: Zohaib Mushtaq. Email:
(This article belongs to the Special Issue: Multimedia Encryption and Information Security)
Computers, Materials & Continua 2024, 79(1), 857-873. https://doi.org/10.32604/cmc.2024.048036
Received 25 November 2023; Accepted 21 February 2024; Issue published 25 April 2024
Abstract
Data security assurance is crucial due to the increasing prevalence of cloud computing and its widespread use across different industries, especially in light of the growing number of cybersecurity threats. A major and ever-present threat is Ransomware-as-a-Service (RaaS) assaults, which enable even individuals with minimal technical knowledge to conduct ransomware operations. This study provides a new approach for RaaS attack detection which uses an ensemble of deep learning models. For this purpose, the network intrusion detection dataset “UNSW-NB15” from the Intelligent Security Group of the University of New South Wales, Australia is analyzed. In the initial phase, the rectified linear unit-, scaled exponential linear unit-, and exponential linear unit-based three separate Multi-Layer Perceptron (MLP) models are developed. Later, using the combined predictive power of these three MLPs, the RansoDetect Fusion ensemble model is introduced in the suggested methodology. The proposed ensemble technique outperforms previous studies with impressive performance metrics results, including 98.79% accuracy and recall, 98.85% precision, and 98.80% F1-score. The empirical results of this study validate the ensemble model’s ability to improve cybersecurity defenses by showing that it outperforms individual MLP models. In expanding the field of cybersecurity strategy, this research highlights the significance of combined deep learning models in strengthening intrusion detection systems against sophisticated cyber threats.Keywords
Cite This Article
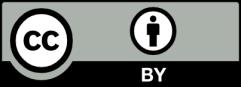
This work is licensed under a Creative Commons Attribution 4.0 International License , which permits unrestricted use, distribution, and reproduction in any medium, provided the original work is properly cited.