Open Access
ARTICLE
Pervasive Attentive Neural Network for Intelligent Image Classification Based on N-CDE’s
Department of Information Systems, Faculty of Computing and Information Technology, King Abdulaziz University, Rabigh, 21911, Saudi Arabia
* Corresponding Author: Anas W. Abulfaraj. Email:
(This article belongs to the Special Issue: Intelligent Management and Machine Learning for Big Data in IoT-Enabled Pervasive Computing)
Computers, Materials & Continua 2024, 79(1), 1137-1156. https://doi.org/10.32604/cmc.2024.047945
Received 23 November 2023; Accepted 04 March 2024; Issue published 25 April 2024
Abstract
The utilization of visual attention enhances the performance of image classification tasks. Previous attention-based models have demonstrated notable performance, but many of these models exhibit reduced accuracy when confronted with inter-class and intra-class similarities and differences. Neural-Controlled Differential Equations (N-CDE’s) and Neural Ordinary Differential Equations (NODE’s) are extensively utilized within this context. N-CDE’s possesses the capacity to effectively illustrate both inter-class and intra-class similarities and differences with enhanced clarity. To this end, an attentive neural network has been proposed to generate attention maps, which uses two different types of N-CDE’s, one for adopting hidden layers and the other to generate attention values. Two distinct attention techniques are implemented including time-wise attention, also referred to as bottom N-CDE’s; and element-wise attention, called top N-CDE’s. Additionally, a training methodology is proposed to guarantee that the training problem is sufficiently presented. Two classification tasks including fine-grained visual classification and multi-label classification, are utilized to evaluate the proposed model. The proposed methodology is employed on five publicly available datasets, including CUB-200-2011, ImageNet-1K, PASCAL VOC 2007, PASCAL VOC 2012, and MS COCO. The obtained visualizations have demonstrated that N-CDE’s are better appropriate for attention-based activities in comparison to conventional NODE’s.Keywords
Cite This Article
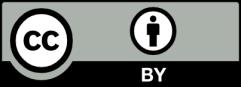